Beyond Single-Cloud AI: Enterprise Lessons from OpenAI Computing Problem
Recent developments at OpenAI have sent ripples through the AI industry, with CEO Sam Altman deciding to look beyond Microsoft for computing power highlighting a critical challenge facing organizations implementing AI: infrastructure scalability. This strategic shift offers valuable lessons for enterprises navigating their own AI journey.
The Computing Power Crisis
The AI landscape is experiencing unprecedented demands on computing infrastructure. OpenAI’s move to explore partnerships beyond Microsoft isn’t just a business decision – it’s a response to a fundamental challenge that organizations of all sizes must ultimately address.
To put this in perspective, training advanced AI models requires massive computing resources:
A single large language model training run can consume the equivalent computing power of thousands of high-end GPUs
Companies may need to update their infrastructure multiple times throughout the development process
Access to computing resources often becomes the critical bottleneck in AI projects
Why Even Tech Giants Struggle
When a company like OpenAI, backed by Microsoft’s vast resources, faces computing constraints, it raises important questions for enterprises building their AI capabilities. The challenge isn’t just about access to resources – it’s about the efficiency and scalability of the entire infrastructure stack.
Key factors driving this situation include:
Exponential growth in model sizes
Increasing complexity of AI applications
Competition for limited chip supplies
Energy consumption concerns
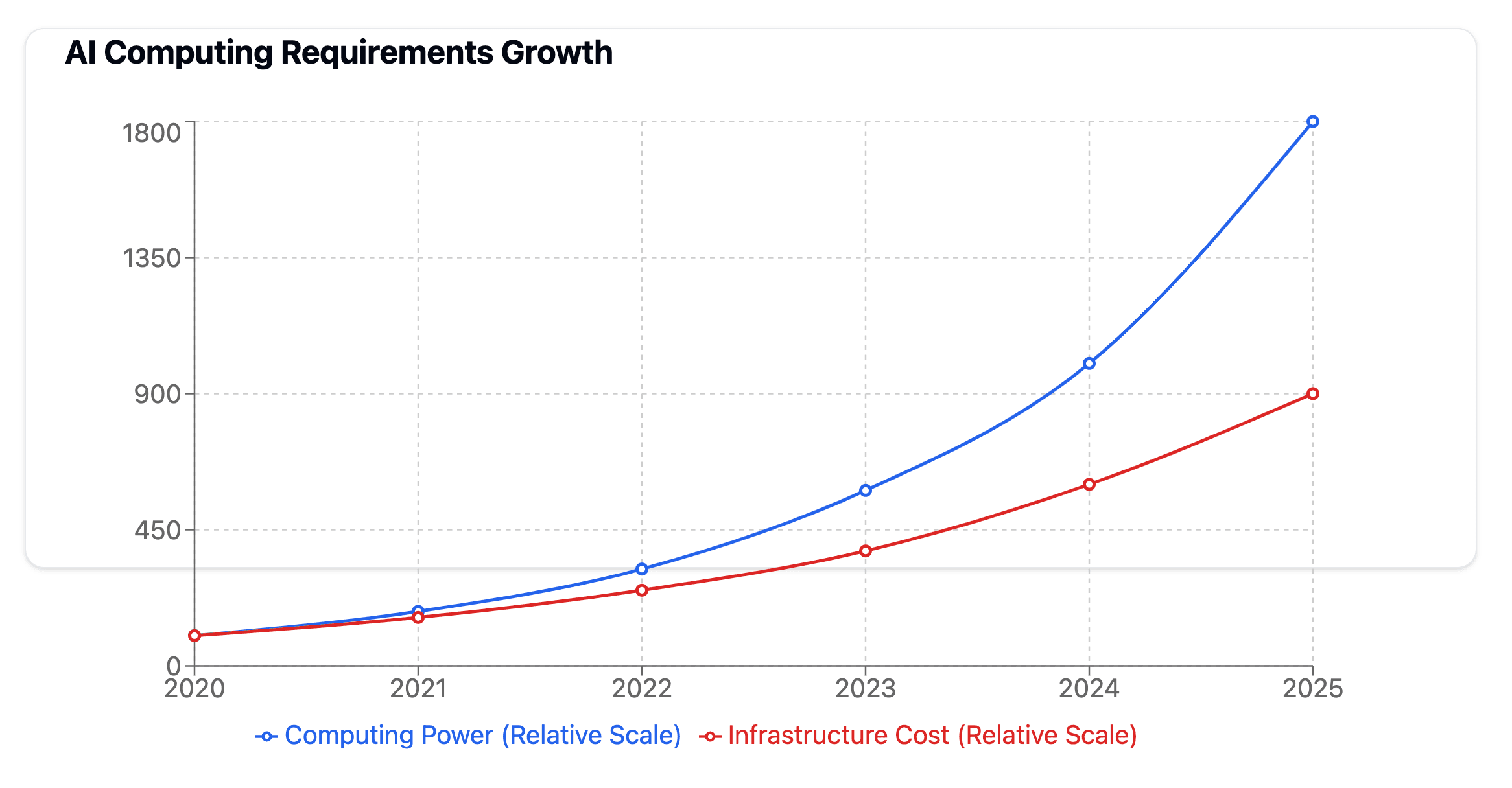
Strategic Infrastructure Decisions
Organizations must take a strategic approach to their AI infrastructure, balancing immediate computing power needs with long-term scalability. The process requires careful consideration of multiple factors that will ultimately shape an organization’s AI capabilities.
Assessment of Current Capabilities
Before making infrastructure decisions, companies need to evaluate their existing computing resources and future requirements. This initial step helps identify potential bottlenecks and areas for improvement. Organizations should focus on understanding their current workloads, projected growth, and specific AI model requirements.
Multi-Vendor Strategy Considerations
Following OpenAI’s lead, enterprises should evaluate the benefits of a multi-vendor approach. This strategy can provide several critical advantages:
Reduced dependency on single providers
Enhanced cost optimization opportunities
Improved resource availability
Stronger negotiating position
Hybrid Infrastructure Planning
The future of enterprise AI infrastructure increasingly points toward hybrid models. These solutions typically combine:
Cloud resources for scalability and flexibility
On-premises computing for sensitive workloads
Edge computing for latency-critical applications
When implementing these strategies, organizations must carefully evaluate their specific needs, taking into account factors such as data security requirements, performance demands, and overall cost structures. The goal is to create a flexible infrastructure that can adapt to changing AI computing demands while maintaining operational efficiency.
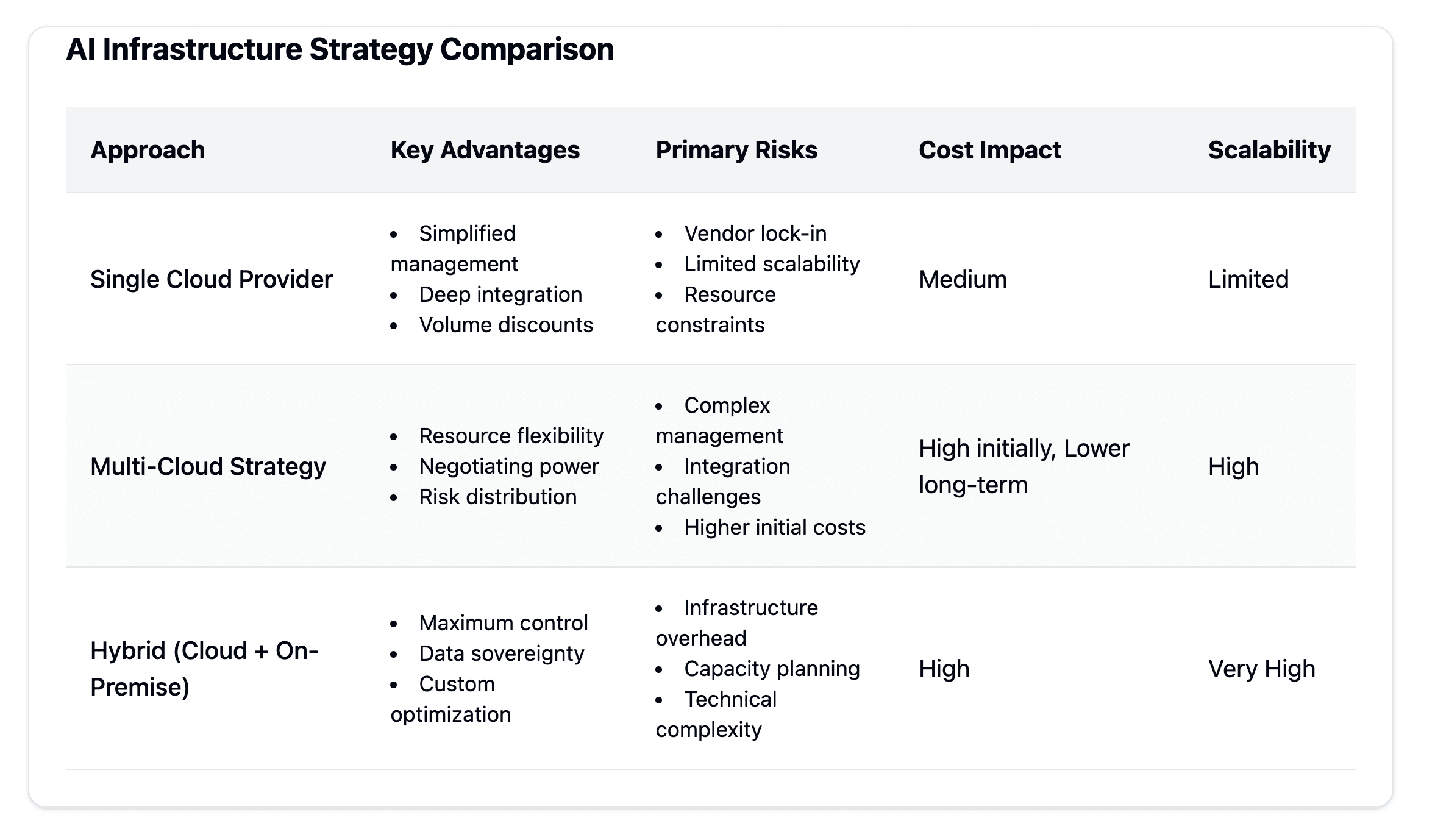
Future-Proofing Enterprise AI
As organizations scale their AI capabilities, future-proofing infrastructure becomes critical for long-term success. The challenges faced by OpenAI computing demonstrate that even companies at the forefront of AI development must constantly update their infrastructure strategy to meet evolving demands.
Today’s AI applications require unprecedented computing power, and this demand will only intensify. Organizations need to develop scalable infrastructure that can adapt to:
Increasing model sizes and complexity
Growing data processing requirements
Expanding business applications
Dynamic workload patterns
The key is building flexibility into your infrastructure strategy while maintaining access to adequate computing resources. This may involve implementing modular systems that can be easily upgraded or expanded as your organization’s AI capabilities mature.
Energy consumption has also emerged as a critical factor in AI infrastructure planning. Organizations must consider:
Power efficiency of computing resources
Cooling system requirements
Sustainable energy sources
Carbon footprint implications
Companies looking to train large AI models should work closely with data center providers who can ultimately help optimize energy usage while maintaining the necessary computing power for their applications.
Recent market developments, including OpenAI’s work on custom chips, highlight the importance of semiconductor strategy. Organizations should:
Diversify hardware suppliers
Consider custom solutions for specific workloads
Maintain relationships with multiple vendors
Plan for potential supply chain disruptions
Action Steps for Organizations
To successfully implement and maintain robust AI infrastructure, organizations should follow a structured approach that aligns with their business goals and capabilities.
Assessment Framework
Begin by evaluating your current position and future needs:
Audit existing computing resources
Map AI project requirements
Analyze skill gaps within your organization
Assess budget constraints and ROI expectations
Implementation Strategy
Develop a phased approach to infrastructure deployment:
Start with pilot projects to test and validate solutions
Scale successful implementations gradually
Monitor performance and adjust as needed
Maintain flexibility for future updates
Risk Mitigation
Protect your organization’s AI investments by:
Implementing redundancy in critical systems
Developing contingency plans for service disruptions
Maintaining detailed documentation of processes
Creating clear escalation procedures
Establishing regular review and update cycles
The path forward requires organizations to take a proactive stance in developing their AI infrastructure. By carefully considering these elements and taking appropriate steps to address them, companies can build a robust foundation for their AI initiatives while remaining adaptable to future developments in the field.
The Bottom Line
As OpenAI’s infrastructure decisions demonstrate, the future of enterprise AI extends beyond relying solely on cloud giants. Organizations must ultimately take a strategic approach to building and scaling their AI infrastructure, carefully balancing computing power requirements with cost considerations and future scalability. Success in this space requires a flexible, multi-faceted strategy that can adapt to rapid technological changes while maintaining operational efficiency.
By taking critical steps today to assess, implement, and future-proof their AI infrastructure, companies can position themselves to fully leverage AI’s transformative capabilities while avoiding the bottlenecks that even industry leaders face. The key is to start the process now, with a clear understanding that the journey to robust AI infrastructure is continuous and evolving.