The Top 4 Enterprise LLM Use Cases with the Best Return on Investment
In the dynamic landscape of modern business, integrating Large Language Models (LLMs) with enterprise data is not just an innovation—it’s a strategic revolution. This fusion of advanced AI technology, exemplified by models like ChatGPT, with the rich data ecosystems of enterprises, is redefining the boundaries of data utility and accessibility.
At Skim AI, we recognize the profound Return on Investment (ROI) this integration offers, specializing in creating custom solutions that streamline operations, enhance decision-making, and unlock new growth potentials.
The impact of connecting enterprise data to LLMs is transformative. It breaks down traditional data silos, allowing departments such as marketing, finance, and human resources to access and interpret data with unprecedented speed and efficiency. This revolution in data management empowers teams to act on real-time insights, fosters strategic workforce planning, and accelerates overall business productivity.
In this blog, we delve into the four top ROI use cases for integrating enterprise data with LLMs, exploring how this strategic move can reshape your business landscape. We’ll examine the transformative potential of FAQ AI workers/agents, the efficiency of Natural Language to SQL for enhanced data analytics, the creative power of AI-driven blog and social media content assistance, and the competitive edge offered by AI workers in sales enablement and contact matching.
1. FAQ AI Workers/Agents for Customer Support
The integration of AI workers or agents, equipped with LLMs, into customer support systems marks a significant stride in the evolution of customer service. These AI-powered agents can automatically and accurately respond to Frequently Asked Questions (FAQs), transforming how businesses interact with their customers.
FAQ AI workers bring a level of efficiency and precision that traditional support systems struggle to match. They are capable of sifting through vast amounts of enterprise data to provide instant and accurate responses to customer queries. This leads to a significant reduction in response times and an increase in customer satisfaction. The cost savings are notable too—by automating responses to common questions, enterprises can reduce the workload on human support staff, thereby cutting down on labor costs and reallocating resources to more complex customer service issues.
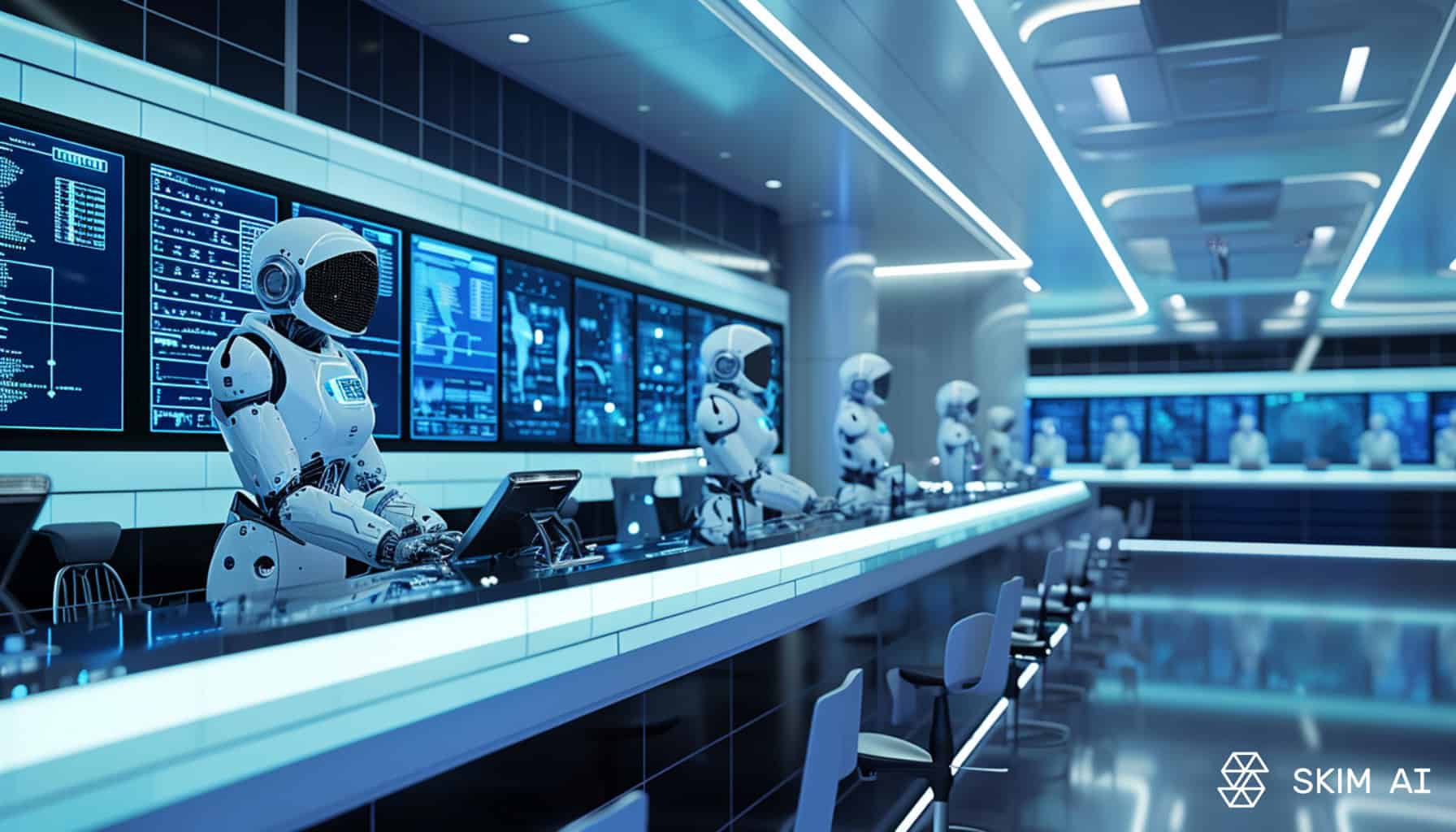
Real-World Use Case: A Global Enterprise Scenario
Imagine a global e-commerce company that receives thousands of customer inquiries daily about product availability, shipping policies, and order tracking. By integrating an LLM-powered FAQ AI worker into their customer support system, they could automate the process of answering these routine queries.
For example, when a customer asks, “How long does shipping take to Canada?”, the AI worker, connected to the company’s logistics database, quickly analyzes the latest shipping timelines and responds with a precise answer like, “Your order will be delivered within 5-7 business days to Canada.” This response is not only immediate but also tailored to the customer’s specific inquiry, enhancing their experience.
Additionally, the AI worker could handle more complex queries by accessing and interpreting detailed enterprise data. For instance, if a customer asks, “I ordered a blue sweater last week, why hasn’t it shipped yet?”, the AI worker can look into the order history, check inventory status, and inform the customer, “The blue sweater is currently backordered, but we expect it to ship by the end of this week.”
Such personalized and efficient handling of customer inquiries not only elevates the customer experience but also frees up human agents to focus on issues that require empathy and nuanced understanding. This blend of AI and human intelligence in customer service represents a significant ROI for the enterprise, both in terms of operational efficiency and enhanced customer relationships.
2. Natural Language to SQL for Enhanced Data Analytics
The integration of LLMs to facilitate natural language processing into SQL queries is a game-changer in the realm of enterprise data analytics. This innovative approach is transforming the traditional data query and analysis process, making it more accessible and intuitive, especially for non-technical staff. It marks a significant shift from complex SQL queries to a more straightforward, natural language approach, democratizing data analytics across various departments in an enterprise.
The application of LLMs for natural language to SQL conversion in enterprise data systems enables a broader range of employees to engage directly with data analytics. This shift is crucial in breaking down the barriers to data access, often encountered in businesses. By simplifying the query process, employees from different departments can now perform data analyses without the need for in-depth SQL knowledge or constant reliance on the IT department. This increased accessibility leads to faster, more informed decision-making processes, significantly enhancing the productivity and efficiency of business operations.
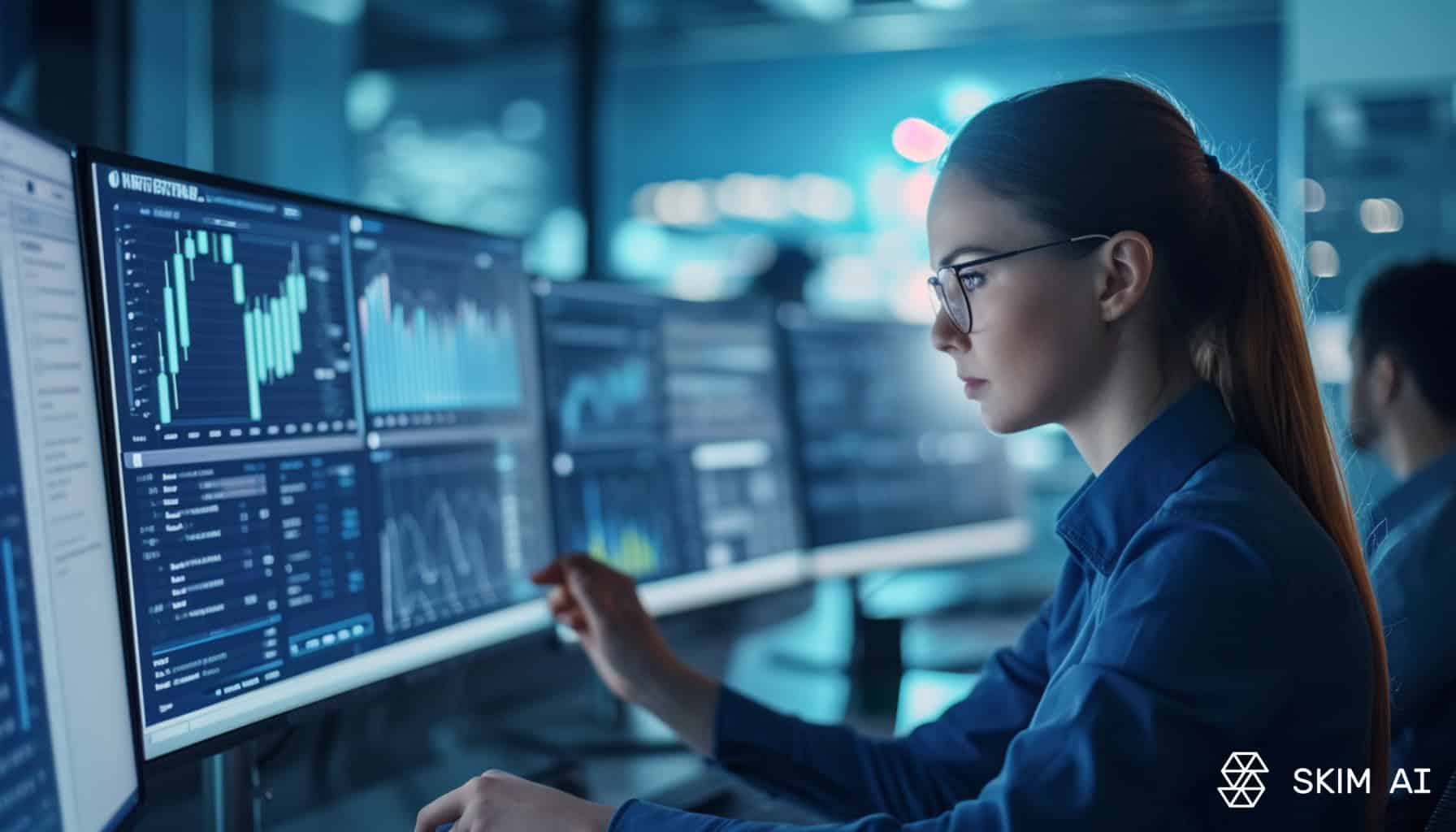
Real-World Use Case: Enhancing Marketing Strategy with Data-Driven Insights
Consider a scenario in a large enterprise where the marketing team needs immediate insights into customer behavior to develop an upcoming campaign strategy. Traditionally, obtaining these insights would require data analysts to formulate and execute complex SQL queries – a process that could be time-consuming. However, with a custom LLM integrated into their enterprise data system, the marketing team can simply submit a natural language query such as, “Show me the purchasing patterns of customers aged 25-35 in the last quarter.”
The LLM seamlessly translates this query into SQL, retrieves the relevant data from the enterprise database, and presents it in an easily interpretable format. The marketing team can quickly identify trends and make data-backed decisions on targeting and campaign design, leading to more effective marketing initiatives. This capability not only expedites the decision-making process but also ensures that marketing strategies are underpinned by real-time, accurate data.
This application of LLMs for natural language to SQL conversion represents a significant productivity gain for the enterprise. It allows teams to harness the power of their business data in making strategic decisions, fostering a culture of data-driven innovation and agility within the organization.
By integrating LLMs for natural language processing into SQL, enterprises not only enhance the quality of their business decisions but also encourage a culture of data-driven innovation and agility.
3. AI-driven blog and Social Media Content Assistance
The integration of LLMs into content creation for blogs and social media is revolutionizing the marketing landscape. By leveraging enterprise data, these AI-driven tools offer unprecedented assistance in generating engaging, relevant, and insight-driven content. This innovation is pivotal for marketing departments, enabling them to create high-quality content more efficiently, resonating with their target audience while saving significant time and resources.
The use of LLMs for blog and social media content creation allows marketing teams to tap into vast reserves of enterprise data, transforming it into compelling narratives and insightful posts. This process goes beyond mere content generation; it involves analyzing customer interactions, market trends, and historical data to produce content that is not only engaging but also strategically aligned with business goals. The result is a more effective marketing approach, with content that speaks directly to the audience’s interests and needs.
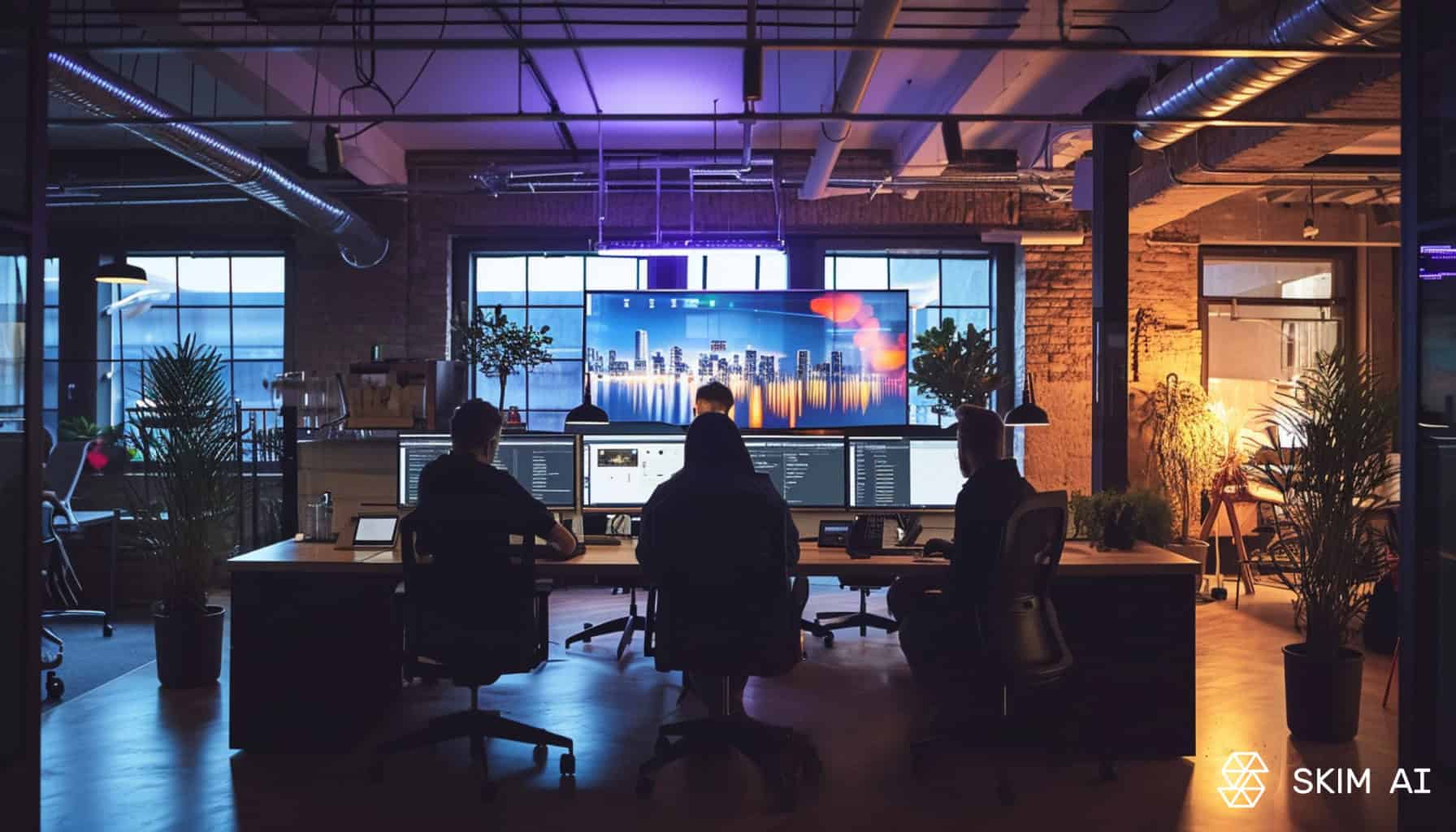
Real-World Use Case: Streamlining Social Media Strategy
Imagine a scenario where a company’s marketing team is planning a social media campaign to increase engagement and drive sales. Traditionally, this task would involve extensive research to understand the audience, brainstorm content ideas, and then craft posts manually – a time-consuming process.
However, with an LLM integrated into their enterprise data system, the team can input a simple natural language query like, “Create social media posts highlighting our most popular products among millennials this season.” The LLM, utilizing the enterprise’s sales and customer interaction data, generates a series of posts that not only feature the most popular products but also include engaging narratives tailored to the millennial audience. The content is data-driven, ensuring relevance and appeal to the target demographic.
This AI-driven approach streamlines the content creation process, enabling the marketing team to focus on strategy and execution rather than getting bogged down in the initial stages of content development. The ability to rapidly produce targeted, data-informed content translates into a more dynamic and responsive social media presence, ultimately leading to increased engagement and sales.
The integration of LLMs for blog and social media content creation represents a significant leap in marketing efficiency and effectiveness. It allows enterprises to leverage their data in new and innovative ways, transforming how they engage with their audience and execute their marketing strategies.
4. AI Workers for Sales Enablement and Contact Matching
Incorporating LLMs into sales enablement processes marks a significant advancement in how sales teams operate and strategize. By analyzing enterprise data, LLMs can significantly enhance lead generation and contact matching, leading to more precise and effective sales strategies. This integration is not just a technological leap; it’s a strategic game-changer that directly contributes to increased conversion rates and more targeted sales outreach.
The application of LLMs in sales revolves around their ability to sift through and analyze extensive enterprise data, identifying potential leads and matching them with the most suitable products or services. This process involves more than just basic data analysis; it includes understanding customer behaviors, preferences, and historical interactions to create a comprehensive profile for targeted outreach.
By leveraging LLMs, sales teams can move beyond traditional lead generation methods. They’re equipped with AI workers that provide insights derived from a deep analysis of customer data, enabling them to tailor their sales pitches and strategies more effectively. This tailored approach not only increases the likelihood of conversions but also enhances customer satisfaction by offering solutions that truly match their needs.
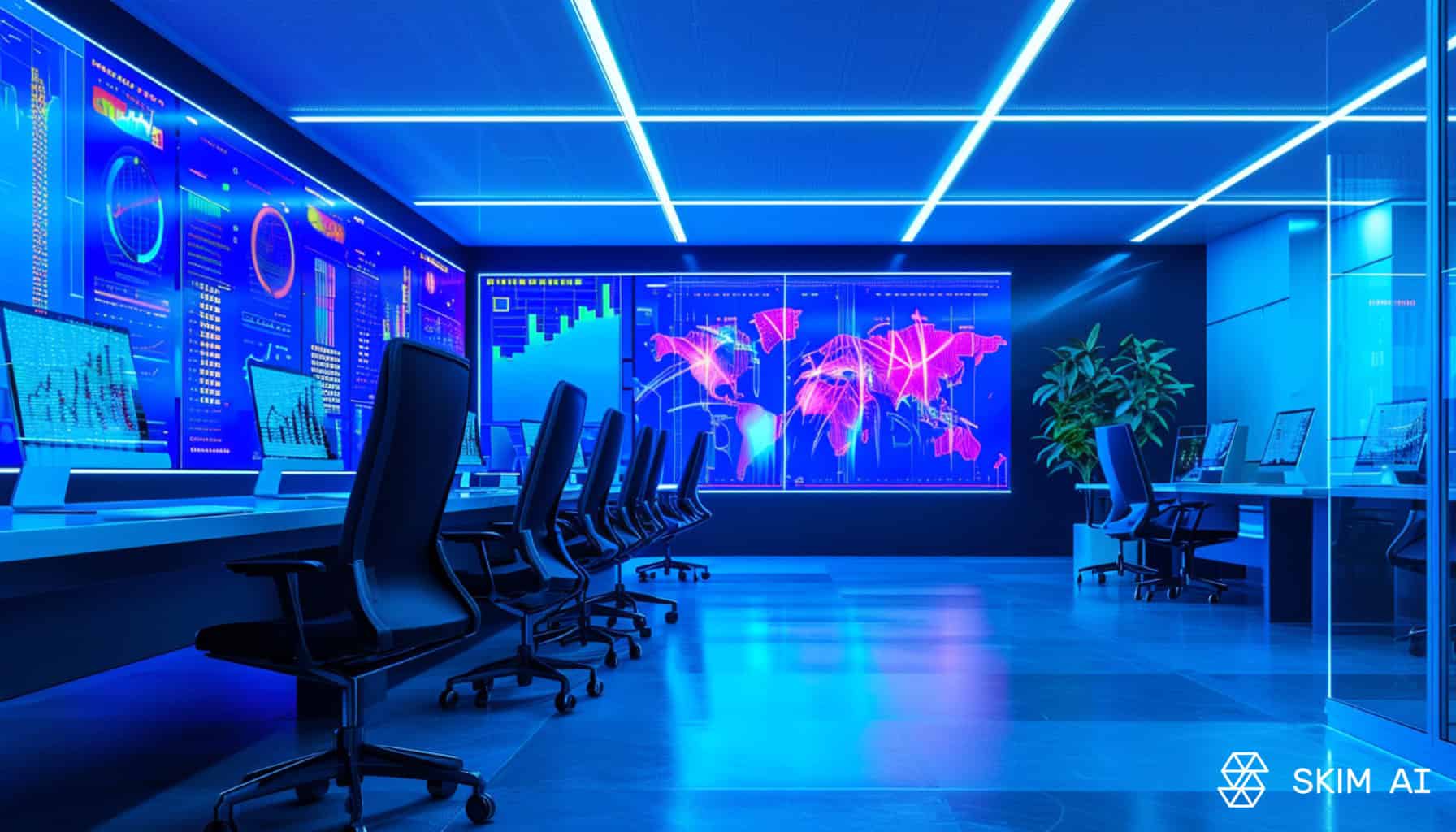
Real-World Use Case: Enhancing Sales Efficiency and Targeting
Consider a software company looking to expand its customer base. Typically, their sales team would spend considerable time researching potential leads, trying to match their software solutions to the needs of various businesses manually. However, with an LLM integrated into their sales process, this task becomes much more efficient and targeted.
For instance, the sales team could use the LLM to analyze enterprise data, including past customer interactions, product preferences, and market trends, to identify potential leads. They could input a query like, “Identify businesses in the healthcare sector that have shown interest in cloud-based solutions in the last six months.” The LLM, drawing on the company’s comprehensive data, would then provide a list of potential leads that fit this specific criterion, along with insights into their particular needs and preferences.
Armed with this information, the sales team can craft personalized pitches, focusing on how their software solutions can address the unique challenges and requirements of these leads. This targeted approach not only saves time but also significantly improves the chances of conversion, as the outreach is based on data-driven insights.
In this way, LLMs redefine sales enablement, turning it into a more strategic, data-informed, and efficient process. The impact on sales strategies and ROI is clear – sales teams can achieve higher conversion rates and more effective outreach, ultimately driving business growth and revenue.
Capitalizing on LLM-Driven Innovations in Enterprises
The integration of LLMs with enterprise data stands as a transformative force in the business world. This series has explored various high-ROI use cases, demonstrating how LLMs can revolutionize customer service, enhance data analytics, streamline content creation, and supercharge sales strategies. By tapping into the potential of LLMs, businesses can access a wealth of data-driven insights, elevating operational efficiency and decision-making processes.
Each use case – from AI workers handling FAQs to LLM-powered blog and social media content assistance – underscores the versatility and impact of LLM integration in enterprises. It’s not merely about adopting a new technology; it’s about embracing a paradigm shift in how data is utilized and decisions are made. The benefits extend beyond improved efficiency and cost savings, fostering a culture of innovation and data-driven strategy.
As we look ahead, the role of LLMs in the business landscape will continue to evolve and expand. Enterprises that effectively harness these AI tools will find themselves at the forefront of their respective industries, equipped with the insights and agility needed to thrive in an increasingly competitive and data-centric world.