10 Mistakes Enterprises Make When Starting an AI Project
The integration of enterprise AI into the business landscape is a transformative endeavor, promising unprecedented innovations and operational efficiencies. However, the journey is intricate and laden with potential pitfalls, as discussed in our previous blog, “10 Reasons Why Enterprise AI Projects Fail.”
In this piece, we delve deeper into the initial mistakes enterprises often make when embarking on machine learning and enterprise AI projects. Avoiding these early mistakes is pivotal for laying a solid foundation for AI projects and ensuring the successful implementation of enterprise AI solutions.
- Mistake 1: Ignoring Data Quality
- Mistake 2: Overlooking Employee Training
- Mistake 3: Underestimating Resource Requirements
- Mistake 4: Setting Ambiguous Goals
- Mistake 5: Lack of Strong Leadership
- Mistake 6: Inadequate Integration with Existing Systems
- Mistake 7: Neglecting Infrastructure Requirements
- Mistake 8: Having Unrealistic Expectations
- Mistake 9: Overlooking the Need for Skilled Data Scientists
- Mistake 10: Ignoring Ethical and Legal Implications
- Navigating the Enterprise AI Journey Successfully
Mistake 1: Ignoring Data Quality
Data is the lifeblood of AI models and machine learning models, serving as the foundational element that powers generative AI and enables it to learn and adapt. High-quality data is crucial for developing accurate and reliable AI models, ensuring the effectiveness of AI applications.
Ignoring the quality of data can lead to the development of flawed AI models, compromising the integrity and reliability of enterprise AI solutions. Poor data quality can result in inaccurate insights and misguided decision-making, affecting the overall success of AI projects and the realization of enterprise AI’s transformative potential.
Mistake 2: Overlooking Employee Training
As enterprise AI continues to evolve, fostering a workforce that is proficient in AI and its applications is non-negotiable. AI literacy among employees is essential for creating an environment conducive to innovation and for leveraging enterprise AI solutions effectively in business processes.
Overlooking the need for employee training in artificial intelligence can hinder the progress of AI projects, leading to the underutilization and misapplication of enterprise AI applications. It can stifle innovation and prevent enterprises from unlocking the full potential of AI in optimizing business processes and driving operational efficiencies.
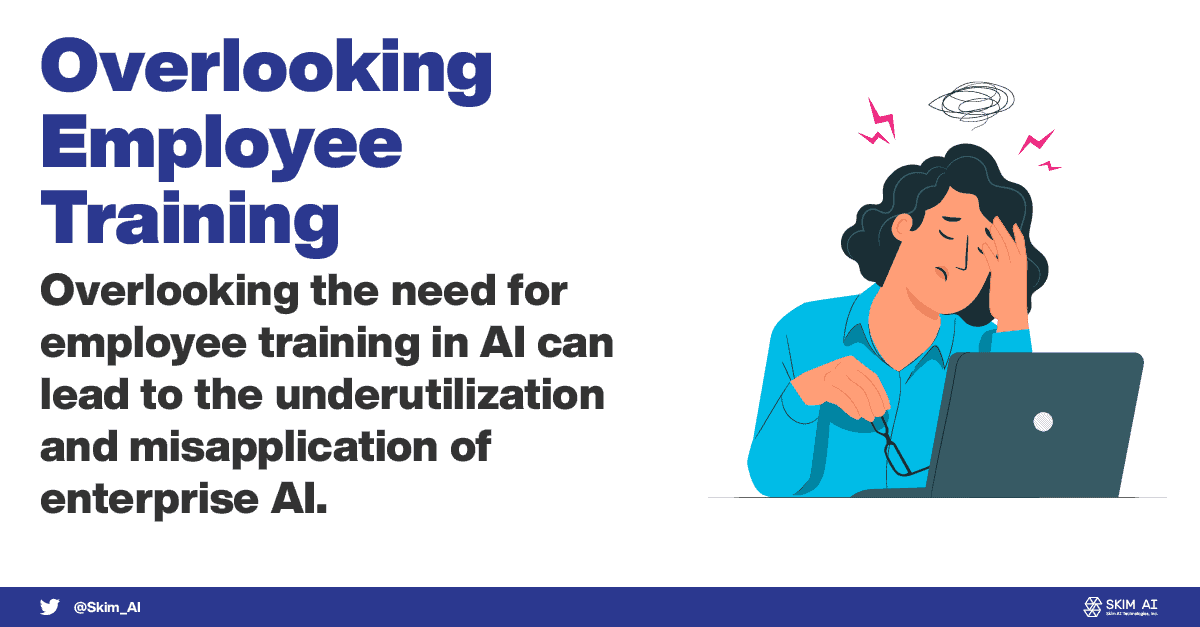
Mistake 3: Underestimating Resource Requirements
Embarking on enterprise AI projects necessitates a comprehensive understanding of the resource requirements. Enterprise AI solutions are intricate, and developing machine learning models that align with business processes can be resource-intensive. A realistic estimation of both time and resources is crucial to avoid overruns and ensure the successful deployment of machine learning and AI models in enterprise software.
Underestimating the resources required can lead to compromised quality and rushed implementations, affecting the success of AI applications. It can strain the enterprise’s resources and can lead to disillusionment with the potential benefits of artificial intelligence, impacting the long-term adoption of enterprise AI.
Mistake 4: Setting Ambiguous Goals
Clear and concise objectives are the cornerstones of successful machine learning and AI projects. They provide the direction and focus needed for aligning artificial intelligence models with business processes and ensuring that enterprise AI initiatives are in sync with the overall business goals.
Setting ambiguous goals can lead to a lack of focus and direction in AI projects, causing misalignments between AI capabilities and business goals. This misalignment can result in project failures, resource wastage, and missed opportunities for innovation and improvement in enterprise AI.
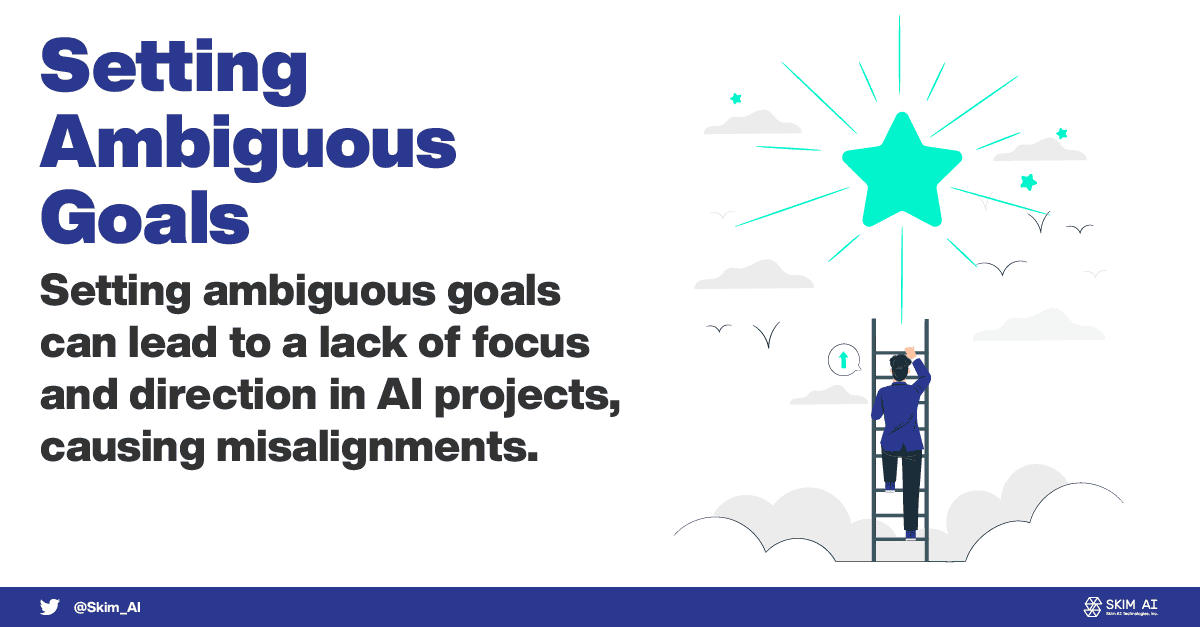
Mistake 5: Lack of Strong Leadership
Effective leadership is pivotal in navigating the complexities of enterprise AI projects. Strong leaders foster innovation, ensure clear communication, and align AI models with the strategic objectives of the enterprise. They play a crucial role in driving AI projects to success and in ensuring the effective implementation of enterprise AI solutions.
A lack of strong leadership can result in project failures, inefficiencies, and a lack of direction and focus in AI projects. It can create ambiguities and a vacuum where the lack of clear guidance can derail enterprise AI initiatives, wasting valuable resources and time.
Mistake 6: Inadequate Integration with Existing Systems
Integrating artificial intelligence models seamlessly with existing systems is crucial for the success of enterprise AI projects. It requires strategic alignment and a thorough understanding of both business processes and enterprise AI applications. Inadequate integration can lead to disjointed AI solutions that do not add value to the enterprise.
Poor integration can lead to ineffective machine learning AI applications, reducing efficiencies and causing disruptions in business processes. It can result in wasted resources and can hinder the advancement and acceptance of enterprise AI in the organizational ecosystem.
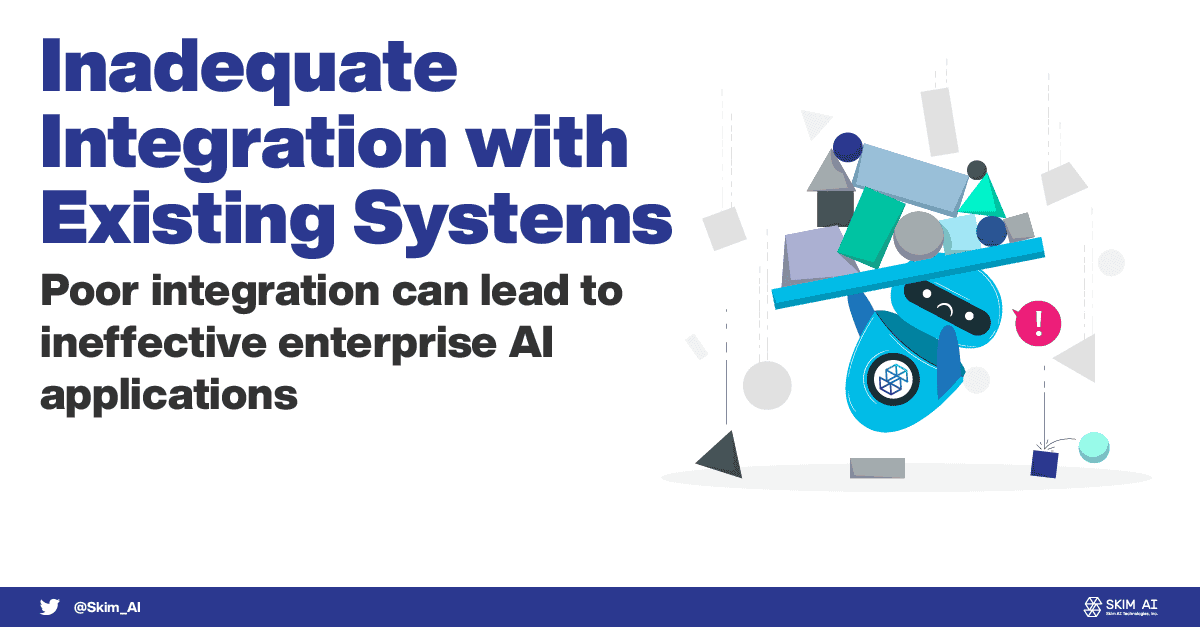
Mistake 7: Neglecting Infrastructure Requirements
A robust, scalable, and flexible technology infrastructure is indispensable for implementing enterprise AI solutions effectively. It supports the complex requirements of AI models and machine learning models, ensuring optimal performance and scalability of enterprise AI applications. Neglecting infrastructure requirements can limit the capabilities and hinder the performance of AI models in enterprise software.
Inadequate technology infrastructure can lead to performance issues, scalability challenges, and limitations in implementing advanced AI models. It can compromise the effectiveness and reliability of enterprise AI applications, leading to project failures and a loss of investment in AI projects.
Mistake 8: Having Unrealistic Expectations
Managing expectations is crucial when implementing enterprise AI. While enterprise AI holds transformative potential, it is essential to understand its limitations and the challenges involved. Unrealistic expectations can lead to disappointment and can tarnish the perception of enterprise AI’s capabilities and benefits in business processes.
Overestimating the capabilities of enterprise AI can lead to project overruns, unmet objectives, and disillusionment with enterprise AI solutions. It can hinder the progress of AI projects and can impact the overall confidence in the deployment of enterprise AI in business operations.
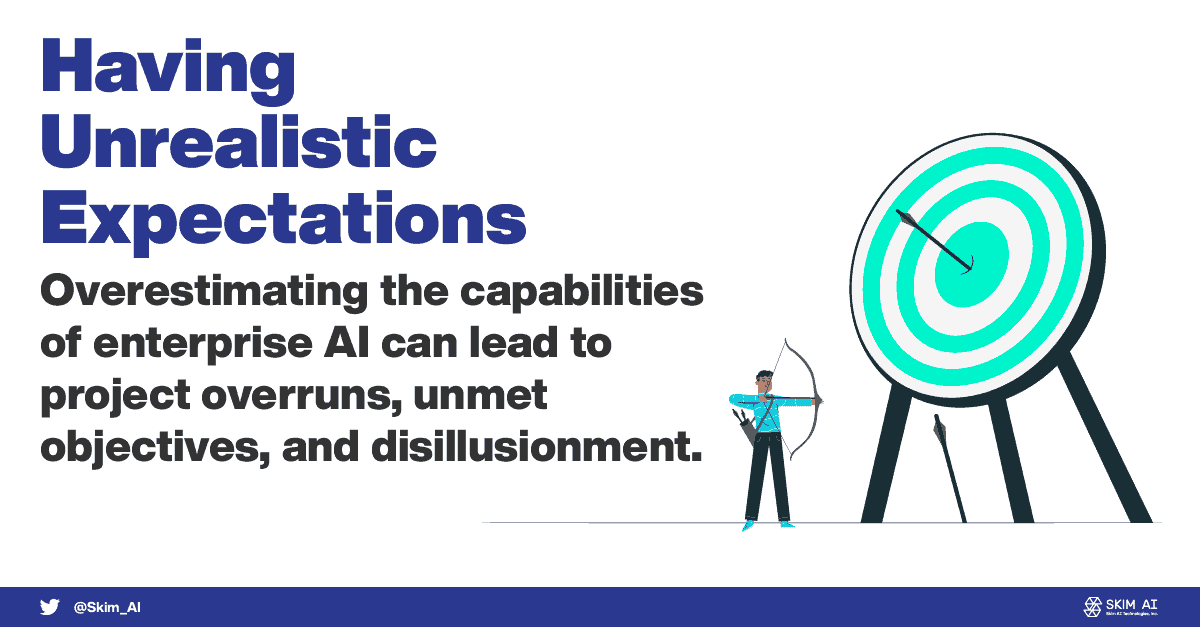
Mistake 9: Overlooking the Need for Skilled Data Scientists
Skilled data scientists are pivotal for developing sophisticated AI models and harnessing the power of machine learning effectively. They bring the necessary expertise and knowledge to AI projects, ensuring the development of innovative and effective enterprise AI solutions. Overlooking the need for skilled data scientists can impede the development and implementation of enterprise AI.
The absence of skilled data scientists can lead to suboptimal development and implementation of enterprise AI applications, affecting the quality and reliability of AI models. It can hinder the advancement and acceptance of enterprise AI, resulting in failed AI projects and unrealized potential.
Mistake 10: Ignoring Ethical and Legal Implications
Addressing ethical concerns and ensuring the responsible use of AI is essential for maintaining trust and credibility in enterprise AI solutions. Ethical considerations and legal implications can pose significant challenges to the implementation of enterprise AI in business processes, and ignoring them can lead to complications and jeopardize AI projects.
Unaddressed ethical and legal concerns can hinder the acceptance and integration of enterprise AI applications, leading to reputational damage and loss of stakeholder trust in enterprise AI. It is crucial to navigate ethical and legal waters responsibly to ensure the successful implementation of enterprise AI.
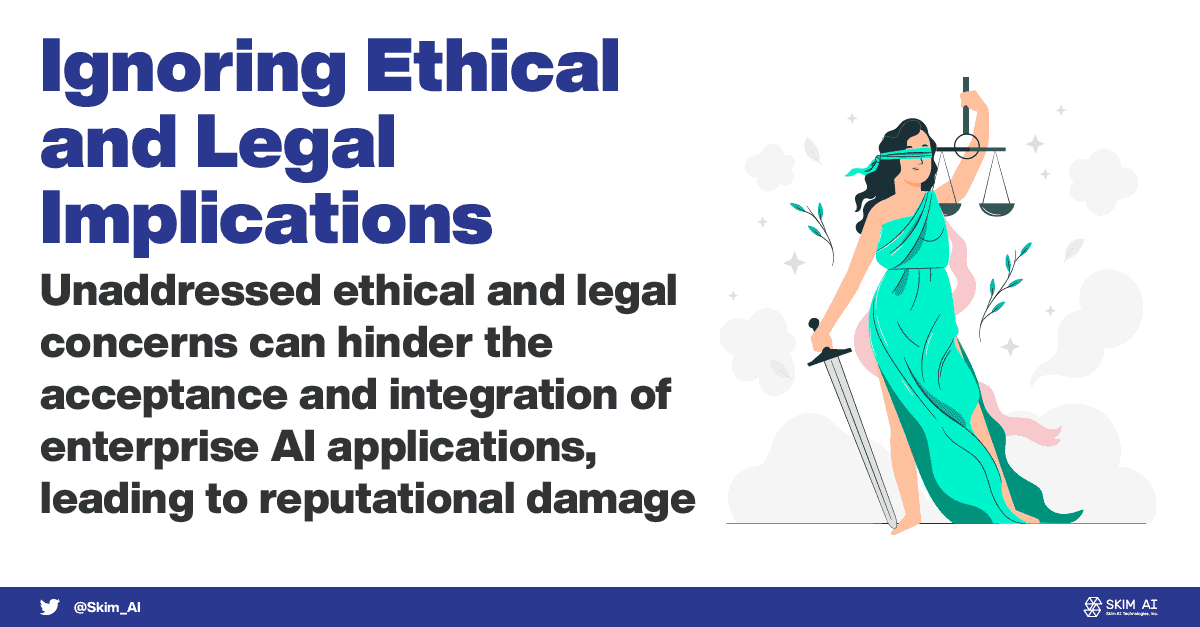
Navigating the Enterprise AI Journey Successfully
Embarking on the journey of implementing enterprise AI is a transformative yet intricate endeavor. It’s a journey filled with potential rewards but also riddled with challenges and pitfalls, as highlighted in our exploration of the common mistakes made in the initial stages of AI projects.
The importance of high-quality data, clear objectives, strong leadership, and robust infrastructure are elements that cannot be overstated. They serve as the pillars upon which successful enterprise AI applications are built.
Neglecting the essential components and overlooking the pivotal aspects such as employee training, integration with existing systems, and the need for skilled data scientists can significantly impede the progress and success of AI projects. It can lead to suboptimal AI models, misalignments with business goals, and a waste of valuable resources and time.
Moreover, managing expectations and addressing the ethical and legal implications are crucial for maintaining trust and credibility in enterprise AI solutions. It is essential to navigate these aspects responsibly and proactively to avoid complications and to ensure the smooth implementation of enterprise AI in business processes.
Avoiding early mistakes and laying a solid foundation are pivotal for unlocking the transformative potential of enterprise AI. It requires a holistic approach, a keen understanding of the intricacies involved, and a strategic alignment with the overall business goals. By addressing the common mistakes and fostering an environment conducive to innovation and progress, enterprises can leverage enterprise AI to redefine their operational strategies and propel themselves into a new frontier of innovation, efficiency, and success.