10 Critical Infrastructure Decisions Every Enterprise Must Make Before Scaling AI
The landscape of enterprise AI computing is evolving rapidly, with recent developments highlighting the complexity of scaling AI infrastructure effectively. As enterprises race to implement AI solutions, the infrastructure decisions made early in the journey can have long-lasting impacts on success, scalability, and cost-effectiveness. Drawing from OpenAI’s recent infrastructure challenges and broader industry experiences, here are the ten critical decisions every organization must carefully consider before scaling their AI initiatives.
- 1. Cloud vs. Hybrid Architecture
- 2. Computing Power Requirements
- 3. Vendor Diversification Strategy
- 4. Cost Optimization Approaches
- 5. Infrastructure Scalability Planning
- 6. Energy Consumption Considerations
- 7. Hardware Procurement Strategies
- 8. Risk Mitigation Tactics
- 9. Performance Monitoring Systems
- 10. Future-Proofing Investments
- The Bottom Line
1. Cloud vs. Hybrid Architecture
The foundation of any enterprise AI strategy begins with a fundamental choice: pure cloud, on-premises, or hybrid infrastructure. This decision shapes not just technical capabilities but the entire trajectory of an organization’s AI journey.
Recent developments, including OpenAI’s strategic shift beyond Microsoft’s infrastructure, highlight why architectural flexibility matters. A hybrid approach often provides the best balance, offering:
Data sovereignty control for sensitive operations
Cost optimization through workload distribution
Reduced vendor lock-in risk
Enhanced operational resilience
For enterprises implementing large language models or other compute-intensive AI applications, the ability to leverage both cloud scalability and on-premises control has become increasingly crucial. This flexibility enables organizations to optimize their infrastructure based on specific workload requirements while maintaining critical data security standards.
2. Computing Power Requirements
Understanding and accurately forecasting computing needs represents a critical challenge in enterprise AI implementation. The rapid evolution of AI models means today’s sufficient computing power might become tomorrow’s bottleneck.
Key considerations for computing requirements include:
Model complexity and training demands
Inference workload patterns
Peak usage management
Growth projection accuracy
Organizations must develop comprehensive assessment frameworks that account for both current operations and future scaling needs. This involves analyzing historical data, understanding model performance requirements, and establishing clear scaling triggers based on business objectives.
3. Vendor Diversification Strategy
OpenAI’s recent move to diversify beyond Microsoft’s infrastructure underscores a crucial lesson for enterprises: over-reliance on a single vendor carries significant risks. A thoughtful multi-vendor strategy enables organizations to:
Maintain negotiating leverage
Ensure service continuity
Access best-in-class capabilities across providers
Optimize costs through competition
However, diversification must be balanced against increased complexity in management and integration. Success lies in finding the right mix of vendors while maintaining operational efficiency through standardized processes and robust integration frameworks.
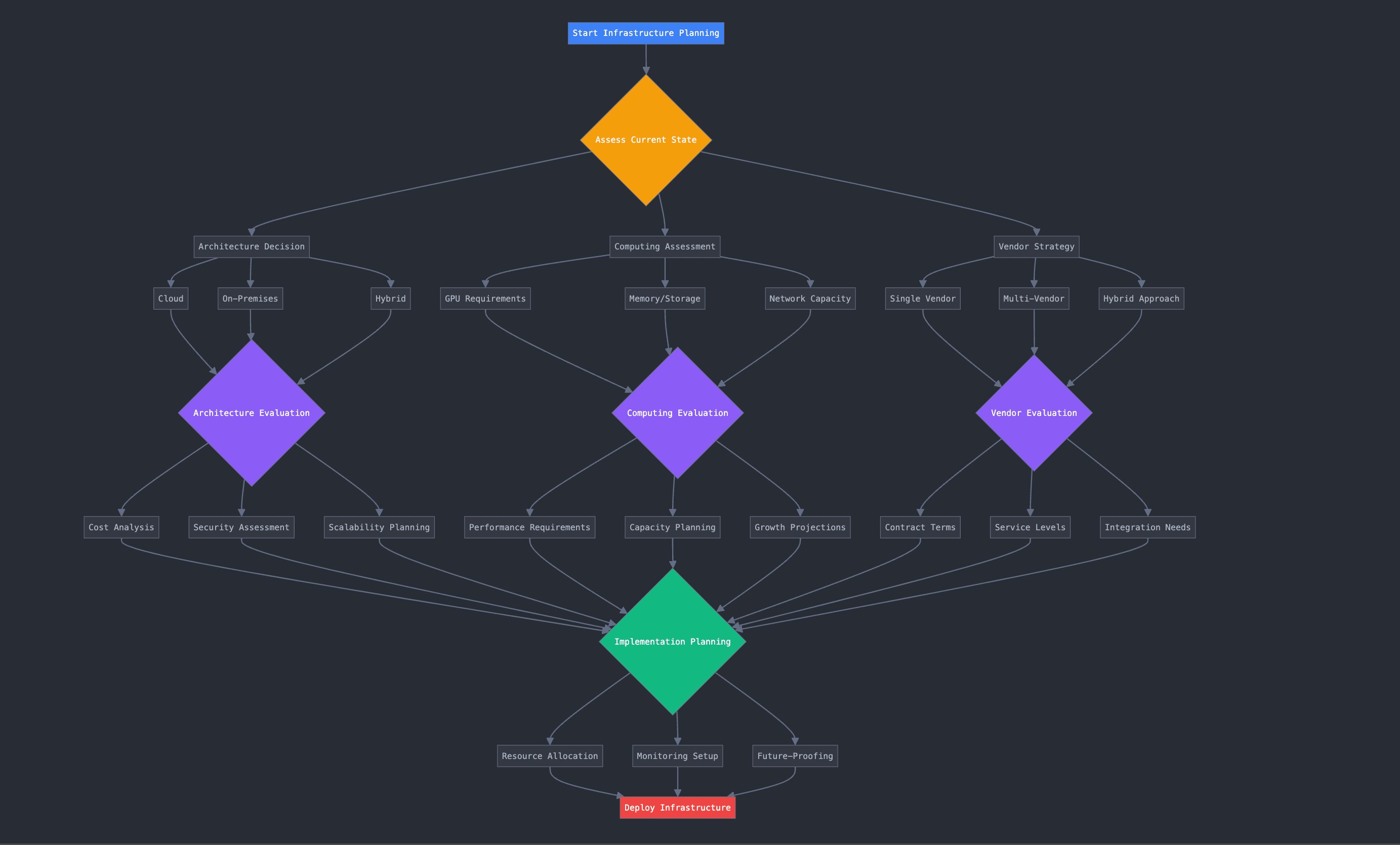
4. Cost Optimization Approaches
Cost management becomes increasingly complex as AI operations scale. OpenAI’s projected $14 billion infrastructure investment by 2026 serves as a stark reminder of how quickly AI computing costs can escalate. Enterprises must establish comprehensive cost optimization strategies from the outset.
Effective cost optimization in AI infrastructure requires:
Clear cost attribution models across business units
Real-time usage monitoring and alerts
Automated resource scaling policies
Regular efficiency audits
Organizations should implement a balanced approach to cost management that doesn’t sacrifice performance or future scalability. This might include utilizing spot instances for non-critical workloads, implementing automatic shutdown policies for development environments, and continuously optimizing model efficiency.
5. Infrastructure Scalability Planning
The ability to scale AI infrastructure efficiently often determines the success or failure of enterprise AI initiatives. Scalability planning must address both technical and operational aspects of growth, ensuring that infrastructure can expand smoothly as demand increases.
Key elements of effective scalability planning include:
Identifying scaling triggers and thresholds
Determining optimal scaling patterns (vertical vs. horizontal)
Planning for geographic distribution
Establishing clear capacity management protocols
Recent industry experiences demonstrate that successful scaling relies not just on technical capabilities but on having clear processes and decision frameworks in place. Organizations must develop scalability roadmaps that align with both technical requirements and business objectives.
6. Energy Consumption Considerations
As AI workloads grow increasingly complex, energy consumption has emerged as a critical consideration for enterprise AI infrastructure. This extends beyond simple cost implications to include environmental impact and sustainability goals.
Organizations must consider:
Power usage effectiveness (PUE) metrics
Cooling system requirements
Carbon footprint implications
Renewable energy options
The financial sector’s experience with AI infrastructure shows that proactive energy management can reduce operational costs by 25-30% while supporting corporate sustainability initiatives. This requires careful planning and ongoing optimization of both hardware and software components to maximize energy efficiency.
7. Hardware Procurement Strategies
Hardware decisions form a critical foundation of AI infrastructure success. With the current global chip shortage and rapid technological advancement, organizations must develop sophisticated procurement strategies that balance immediate needs with long-term flexibility.
Strategic hardware procurement requires:
Clear refresh cycle planning
Vendor evaluation frameworks
Supply chain risk assessment
Performance benchmarking standards
The key is maintaining flexibility while ensuring access to critical resources. Organizations should consider a mix of owned hardware and flexible resources, similar to how OpenAI combines custom chips with vendor solutions.
8. Risk Mitigation Tactics
As AI becomes increasingly central to business operations, robust risk mitigation strategies become essential. Recent industry experiences highlight the importance of comprehensive risk management approaches that address both technical and operational vulnerabilities.
Essential risk mitigation elements include:
Redundancy planning across critical systems
Geographic distribution of resources
Regular disaster recovery testing
Security protocol implementation
Compliance framework adherence
9. Performance Monitoring Systems
Effective monitoring systems provide the visibility needed to maintain optimal AI infrastructure performance. Organizations must implement comprehensive monitoring solutions that track both technical metrics and business KPIs.
Key monitoring considerations include:
Real-time performance tracking
Predictive maintenance capabilities
Capacity utilization metrics
Cost efficiency indicators
User experience monitoring
10. Future-Proofing Investments
The rapid pace of AI advancement requires organizations to balance current needs with future flexibility. Future-proofing involves strategic decisions about technology adoption, upgrade paths, and infrastructure evolution.
Critical aspects include:
Technology roadmap development
Upgrade path planning
Integration flexibility
Innovation capacity building
The Bottom Line
As enterprises continue their AI journey, these ten infrastructure decisions form the foundation for successful scaling and sustainable growth. The experiences of industry leaders like OpenAI demonstrate that thoughtful planning and strategic decision-making in these areas can mean the difference between successful AI implementation and costly setbacks. Organizations that carefully consider and address these critical factors while maintaining flexibility for future evolution will be best positioned to capitalize on AI’s transformative potential.