Як викликати OpenAI o1 + чи варто його використовувати? - AI&YOU #72
Статистика тижня: o1 has shown exceptional skill, ranking in the 89th percentile on Codeforces, a renowned platform for coding challenges. (OpenAI)
OpenAI’s new o1 model marks a paradigm shift in how AI processes and responds to complex queries. Unlike its predecessors, o1 is designed to “think” through problems before generating a response, mimicking a more human-like reasoning process. This fundamental change in model architecture necessitates a corresponding evolution in our prompting techniques.
У цьому випуску AI&YOU ми ділимося думками з трьох блогів, які ми опублікували на цю тему:
- How to Prompt OpenAI o1 + Should You Use It? – AI&YOU #72
- Розуміння можливостей міркувань o1
- Внутрішній ланцюжок міркувань
- Performance Leaps in Complex Tasks
- Ключові принципи надання підказки o1
- Простота і прямота у підказках
- Уникнення надмірних вказівок
- Використання роздільників для більшої ясності
- How to Optimize Input for o1
- Хто повинен використовувати o1-модель OpenAI?
- Ідеальні кандидати для усиновлення o1
- 15 Stats/Facts to Know About OpenAI’s o1 Model
- Підсумок
- Дякуємо, що знайшли час прочитати AI & YOU!
How to Prompt OpenAI o1 + Should You Use It? – AI&YOU #72
For AI enterprises and developers accustomed to working with previous models like GPT-4o, adapting to o1’s unique characteristics is crucial. The prompting strategies that yielded optimal results with earlier models may not be as effective—or could even hinder performance—when applied to o1.
Understanding how to effectively prompt this new model is key to unlocking its full potential and leveraging its advanced reasoning capabilities in real-world applications.
Розуміння можливостей міркувань o1
Хоча такі моделі, як GPT-4o, чудово генерували текст, подібний до людського, і виконували широкий спектр мовних завдань, вони часто мали проблеми зі складними міркуваннями, особливо в галузях, що вимагають логічного покрокового розв'язання проблем. Однак модель o1 була спеціально розроблена, щоб заповнити цю прогалину.
Ключова відмінність полягає в тому, як o1 обробляє інформацію. На відміну від попередніх моделей, які генерують відповіді на основі розпізнавання шаблонів у навчальних даних, o1 використовує більш структурований підхід до вирішення проблем. Це дозволяє йому вирішувати завдання, які вимагають багатокрокових міркувань, логічних висновків і навіть творчого підходу, зі значно підвищеною точністю.
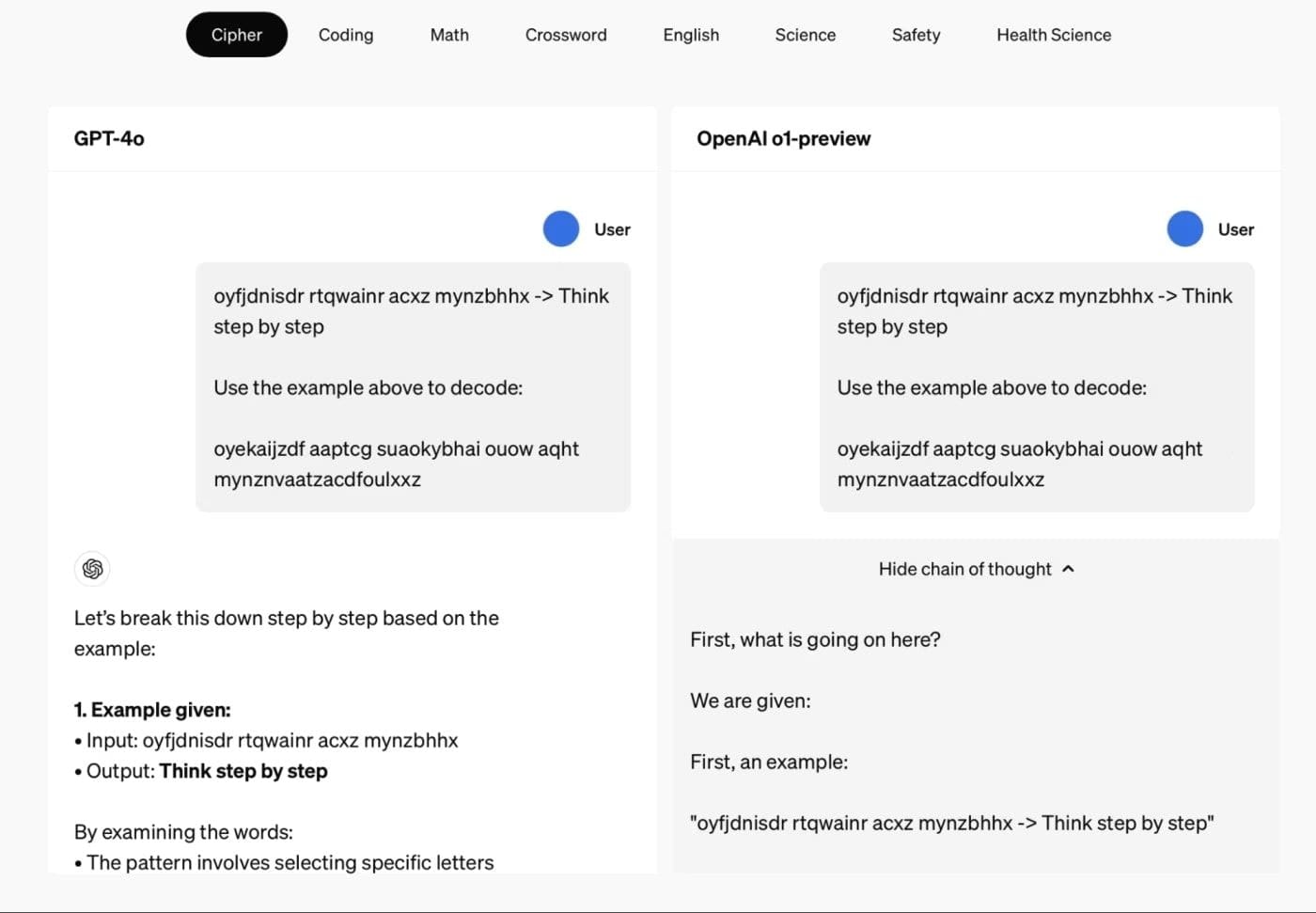
Внутрішній ланцюжок міркувань
В основі можливостей o1 лежить інтегрований ланцюжок думок (CoT) міркувань. Цей підхід, який раніше використовувався як зовнішня техніка підказок, тепер вбудований безпосередньо в архітектуру моделі. Коли o1 отримує складний запит, він не відразу генерує відповідь. Замість цього він спочатку розбиває проблему на менші, керовані кроки.
Цей внутрішній процес міркувань дозволяє o1:
Визначте ключові компоненти проблеми
Встановлення логічних зв'язків між різними елементами
Розглянемо кілька підходів до вирішення завдання
Оцінювати та коригувати власні міркування в міру їхнього розвитку
Performance Leaps in Complex Tasks
o1’s integration of CoT reasoning has led to remarkable improvements in complex logical tasks:
Розв'язання математичних задач: Achieves accuracy levels orders of magnitude higher than predecessors on olympiad-level problems.
Coding capabilities: Rivals skilled human programmers in software development and debugging.
Наукова аргументація: Excels in data analysis and hypothesis generation, opening new research frontiers.
Багатокроковий логічний висновок: Handles tasks requiring complex step-by-step reasoning with increased proficiency.
By integrating CoT reasoning, o1 has achieved substantial improvements in tasks demanding complex cognition, setting new benchmarks in AI capabilities.
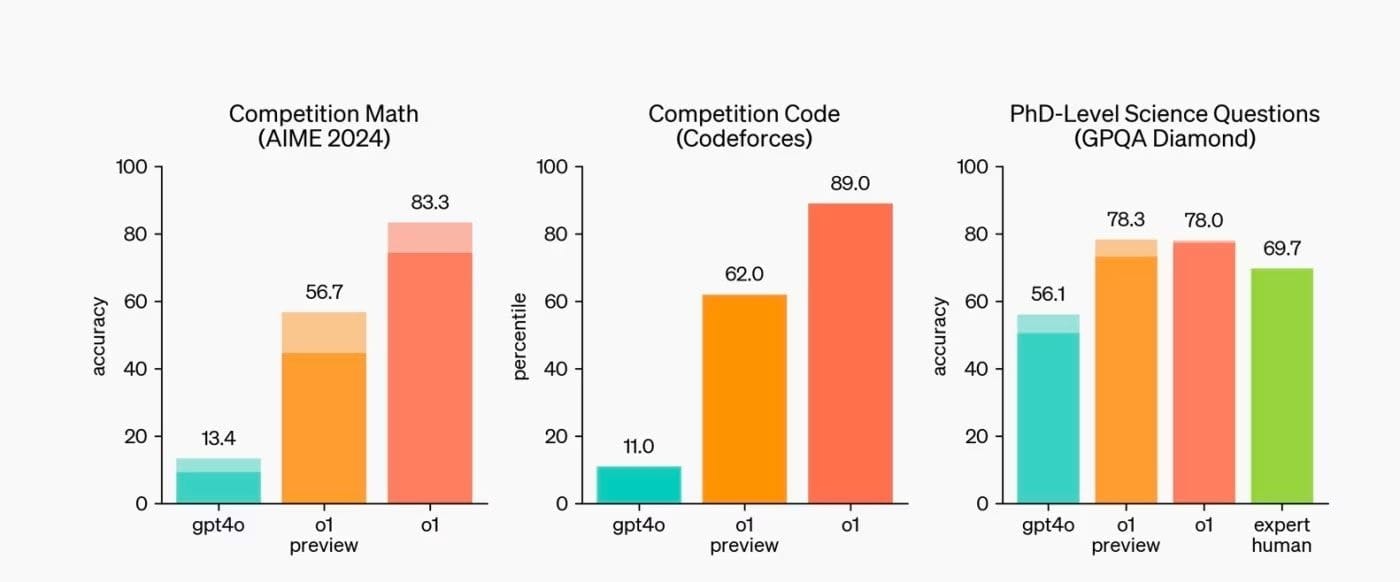
Ключові принципи надання підказки o1
Заглиблюючись у мистецтво підказки моделі o1 OpenAI, важливо розуміти, що це нове покоління моделей міркувань вимагає зміни нашого підходу. Давайте розглянемо ключові принципи, які допоможуть вам використовувати весь потенціал розширених можливостей o1.
Простота і прямота у підказках
When it comes to prompting o1, simplicity is key. Unlike previous models that often benefited from detailed instructions or extensive context, o1’s built-in reasoning capabilities allow it to perform best with straightforward prompts.
Ось кілька порад щодо створення простих і прямих підказок:
Будьте чіткими та лаконічними: Сформулюйте своє питання або завдання прямо, без зайвих подробиць.
Уникайте надмірних пояснень: Довіряйте здатності моделі розуміти контекст і виводити деталі.
Зосередьтеся на основній проблемі: Представте основні елементи вашого запиту без зайвої інформації.
Наприклад, замість того, щоб надавати покрокові інструкції для розв'язання складної математичної задачі, ви можете просто констатувати: "Розв'яжіть рівняння і поясніть свої міркування: 3x^2 + 7x - 2 = 0".
Уникнення надмірних вказівок
While previous models often benefited from detailed instructions or examples (a technique known as “few-shot learning”), o1’s improved performance and internal reasoning process make such guidance less necessary and potentially counterproductive.
Розглянемо наступне:
Не намагайтеся наводити багато прикладів або розлогий контекст, якщо це не є абсолютно необхідним.
Дозвольте моделі використовувати власні можливості міркування, а не намагайтеся керувати її розумовим процесом.
Уникайте чіткого зазначення кроків або методів розв'язання проблеми, оскільки це може порушити внутрішній ланцюжок міркувань o1.
Утримуючись від надмірних вказівок, ви дозволяєте o1 повною мірою використовувати свої передові моделі міркувань і потенційно знаходити більш ефективні або інноваційні рішення для складних завдань міркувань.
Використання роздільників для більшої ясності
Хоча простота має вирішальне значення, бувають випадки, коли вам потрібно надати структуровані дані або відокремити різні компоненти вашого запиту. У таких випадках використання роздільників може значно підвищити чіткість і допомогти o1 ефективніше опрацювати ваші дані.
Розмежувачі слугують кільком цілям:
Вони чітко розділяють різні частини вашого запиту.
Вони допомагають моделі розрізняти інструкції, контекст і власне запит.
Їх можна використовувати для позначення певних форматів або типів інформації.
Деякі ефективні способи використання роздільників включають в себе наступні:
Потрійні лапки: """Ваш текст тут"""
Теги у стилі XML: Ваша інструкція тут</instruction
Тире або зірочки: - або ***
Чітко позначені розділи: [КОНТЕКСТ], [ЗАПИТ], [ФОРМАТ ВИВОДУ].
Наприклад, під час роботи з даними секвенування клітин або іншою науковою інформацією ви можете структурувати підказку таким чином:
—
[КОНТЕКСТ]
Нижче наведено набір даних з експерименту з секвенування клітин:
<data>
...ваші дані тут...
</data
[QUERY]
Проаналізуйте ці дані та визначте будь-які значущі закономірності чи аномалії.
[ВИХІДНИЙ ФОРМАТ].
Надайте свій аналіз у вигляді структурованого звіту з розділами "Методи", "Результати" та "Висновки".
—
Ефективно використовуючи роздільники, ви можете забезпечити необхідний контекст і структуру, не перевантажуючи міркування o1 і не втручаючись у його внутрішній ланцюжок мислення.
How to Optimize Input for o1
Effectively leveraging o1’s advanced reasoning capabilities requires optimized input. Balance context and conciseness by providing essential background without overwhelming the model. Focus on quality over quantity, trusting o1’s ability to infer and reason. For complex tasks, offer a brief overview rather than an exhaustive explanation.
When using Розширене покоління пошуку (RAG) with o1, be selective with external information. Prioritize high-quality, relevant data over volume, using RAG primarily for specific facts rather than general context. This targeted approach enhances o1’s performance on domain-specific tasks without overwhelming its reasoning process.
Embrace o1’s improved performance by trusting it with more challenging, nuanced prompts. Expect sophisticated responses even from concise inputs, and experiment with complex queries that might have been unsuitable for previous AI models. This adaptation allows you to fully harness o1’s potential for complex reasoning tasks.
Хто повинен використовувати o1-модель OpenAI?
Оскільки підприємства та дослідники стикаються з дедалі складнішими викликами та появою нових моделей LLM, виникає питання: чи варто використовувати OpenAI o1 для моїх конкретних потреб?
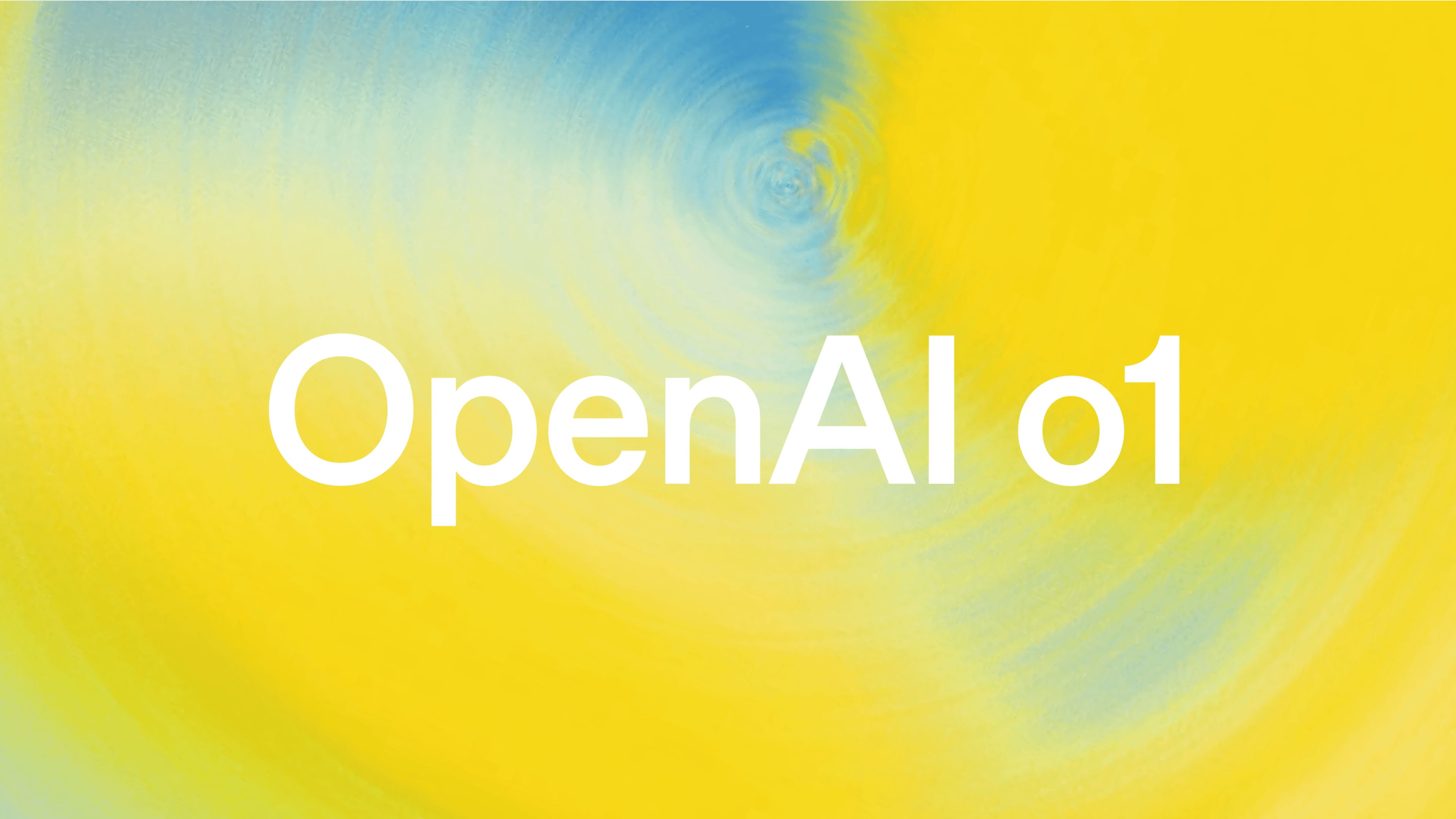
Ідеальні кандидати для усиновлення o1
Розглядаючи питання про те, кому слід використовувати модель o1 OpenAI, ми виділили кілька груп, які особливо добре підходять для використання її розширених можливостей. Унікальні сильні сторони моделі o1 в комплексних міркуваннях і вирішенні проблем роблять її безцінним інструментом для тих, хто працює на передовій інновацій та відкриттів.
1️⃣ Research and Development Teams: R&D teams across industries should adopt o1 for its ability to tackle complex challenges using chain of thought reasoning. This model can accelerate research processes, from drug discovery to experimental design, by efficiently analyzing complex interactions and generating hypotheses. O1’s capacity for detailed, step-by-step reasoning aligns well with R&D’s rigorous approach, making it an invaluable tool for exploring new research directions and solving multi-step problems.
2️⃣ Software Development and Coding: o1’s enhanced abilities in tackling coding tasks, optimizing algorithms, and debugging complex systems make it an invaluable asset for developers. For competitive programmers, o1’s systematic approach to coding challenges mirrors top-tier programmers’ thought processes, serving not just as a tool but as a potential mentor to improve problem-solving skills.
3️⃣ Scientific and Academic Institutions: In scientific research and academia, o1’s advanced reasoning capabilities excel at analyzing vast datasets, formulating hypotheses, and suggesting experimental approaches across fields from astrophysics to genomics. Its ability to provide detailed explanations for complex concepts makes it a powerful aid in both research and education. In theoretical physics and advanced mathematics, o1’s proficiency could lead to new insights on long-standing questions, making it an essential tool for pushing the boundaries of human knowledge.
15 Stats/Facts to Know About OpenAI’s o1 Model
1️⃣ 83% accuracy on International Mathematics Olympiad qualifier
This is a significant improvement over GPT-4o’s 13%, showcasing o1’s advanced mathematical reasoning abilities.
2️⃣ 89th percentile ranking on Codeforces
Demonstrates o1’s exceptional skill in competitive programming and solving complex algorithmic problems.
3️⃣ 74% success rate on AIME problems
A huge leap from GPT-4o’s 9%, highlighting o1’s prowess in tackling difficult, multi-step mathematical challenges.
4️⃣ PhD-level accuracy on GPQA benchmark for physics, biology, and chemistry
Shows o1’s versatility across scientific disciplines, making it valuable for high-level scientific research.
5️⃣ 128,000 token context window
Allows o1 to process and understand much longer pieces of text or more complex problems in a single prompt.
6️⃣ Two variants: o1-preview and o1-mini
Offers flexibility for different use cases, balancing capability and speed.
7️⃣ Uses internal “reasoning tokens” for problem-solving
Enables o1 to break down complex problems into steps, mimicking human-like reasoning.
8️⃣ Improved performance in challenging languages like Yoruba and Swahili
Enhances o1’s utility for multilingual tasks and global applications.
9️⃣ 0.44 score on SimpleQA test for hallucinations
Lower than GPT-4o’s 0.61, indicating reduced likelihood of generating false information.
🔟 94% correct answer selection on unambiguous questions
Improvement over GPT-4o’s 72%, suggesting enhanced fairness and reduced bias in responses.
1️⃣1️⃣ Enhanced jailbreak resistance and content policy adherence
Improves safety and reliability for public-facing or sensitive applications.
1️⃣2️⃣ Slower response times compared to previous models
Trade-off for its more extensive reasoning processes and deeper analysis capabilities.
1️⃣3️⃣ o1-preview pricing: $15 per million input tokens, $60 per million output tokens
Reflects the advanced capabilities and increased computational resources required.
1️⃣4️⃣ Excels in mathematics, coding, and scientific reasoning
Shows particular excellence in STEM fields, making it invaluable for research institutions, tech companies, and educational organizations.
1️⃣5️⃣ o1-mini priced at $3 per million input tokens
Offers a more cost-effective option compared to o1-preview, though likely with some trade-offs in capability.
Підсумок
OpenAI’s o1 model represents a significant leap forward in AI capabilities, particularly in complex reasoning tasks across STEM fields. Its improved performance in areas like mathematics, coding, and scientific analysis, coupled with enhanced safety features and reduced biases, makes it a powerful tool for enterprises tackling sophisticated challenges.
However, the trade-offs in terms of processing speed and higher costs necessitate careful consideration. As AI continues to evolve, o1 stands as a testament to the rapid advancements in the field, offering unprecedented capabilities that could potentially transform how businesses and researchers approach complex problem-solving in the near future.
Дякуємо, що знайшли час прочитати AI & YOU!
Щоб отримати ще більше матеріалів про корпоративний ШІ, включаючи інфографіку, статистику, інструкції, статті та відео, підписуйтесь на канал Skim AI на LinkedIn
We enable Venture Capital and Private Equity backed companies in the following industries: Medical Technology, News/Content Aggregation, Film & Photo Production, Educational Technology, Legal Technology, Fintech & Cryptocurrency to automate work and scale with AI.