LLM을 위한 몇 샷 프롬프트, 학습 및 미세 조정 - AI&YOU #67 LLM을 위한 몇 샷 프롬프트, 학습 및 미세 조정 - AI&YOU #67
- Few-Shot Prompting, Learning, and Fine-Tuning for LLMs – AI&YOU #67 Few-Shot Prompting, Learning, and Fine-Tuning for LLMs – AI&YOU #67
- Few-Shot Prompting, Learning, and Fine-Tuning for LLMs – AI&YOU #67
- AI의 데이터 부족 문제
- 몇 번의 샷 학습 대 기존의 지도 학습
- 효율적인 샘플 학습의 스펙트럼
- Few Shot Prompting vs Fine Tuning LLM
- 몇 번만 촬영하면 됩니다: LLM 잠재력 발휘하기
- LLM 미세 조정: 제한된 데이터로 모델 조정하기
- 소수의 샷 프롬프트 대 미세 조정: 올바른 접근 방식 선택하기
- Top 5 Research Papers for Few-Shot Learning
- 결론
- 시간을 내어 AI & YOU를 읽어주셔서 감사합니다!
Few-Shot Prompting, Learning, and Fine-Tuning for LLMs – AI&YOU #67 Few-Shot Prompting, Learning, and Fine-Tuning for LLMs – AI&YOU #67
금주의 통계: Research by MobiDev on few-shot learning for coin image classification found that using just 4 image examples per coin denomination, they could achieve ~70% accuracy.
In AI, the ability to learn efficiently from limited data has become crucial. That’s why it’s important for enterprises to understand few-shot learning, few-shot prompting, and fine-tuning LLMs.
이번 주 AI&YOU에서는 해당 주제에 대해 게시한 세 개의 블로그에서 얻은 인사이트를 살펴보고자 합니다:
Few-Shot Prompting, Learning, and Fine-Tuning for LLMs – AI&YOU #67
Few Shot Learning is an innovative machine learning paradigm that enables AI models to learn new concepts or tasks from only a few examples. Unlike traditional supervised learning methods that require vast amounts of labeled training data, Few Shot Learning techniques allow models to generalize effectively using just a small number of samples. This approach mimics the human ability to quickly grasp new ideas without the need for extensive repetition.
몇 샷 학습의 핵심은 사전 지식을 활용하고 새로운 시나리오에 빠르게 적응하는 능력에 있습니다. 모델이 '학습하는 방법'을 배우는 메타 학습과 같은 기술을 사용하여 Few Shot Learning 알고리즘은 최소한의 추가 교육으로 다양한 작업을 처리할 수 있습니다. 이러한 유연성 덕분에 데이터가 부족하거나 비용이 많이 들거나 지속적으로 진화하는 시나리오에서 매우 유용한 도구가 될 수 있습니다.
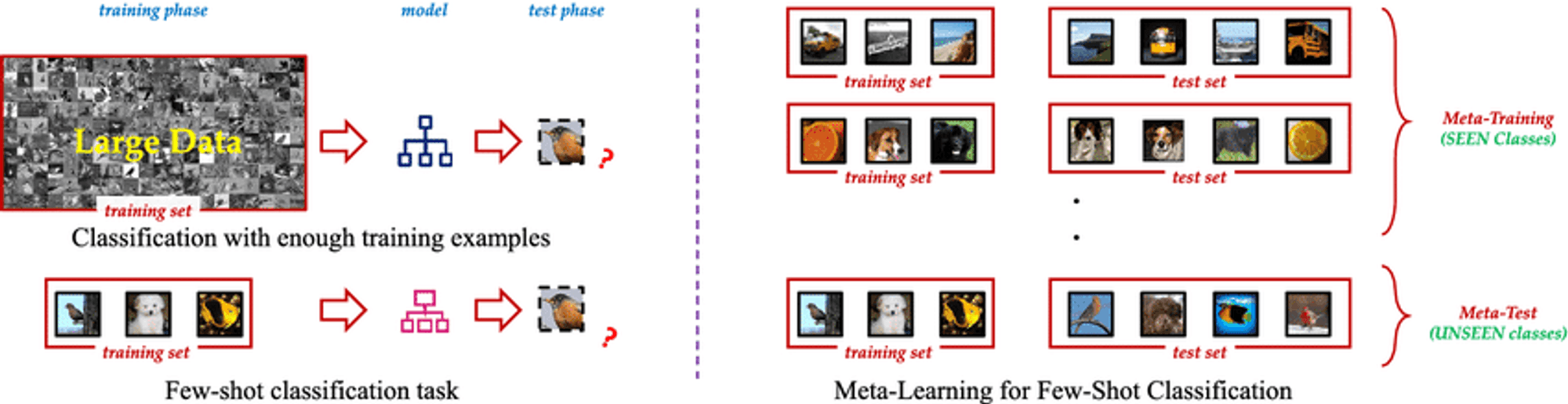
AI의 데이터 부족 문제
모든 데이터가 똑같이 생성되는 것은 아니며, 고품질의 라벨링된 데이터는 희귀하고 귀중한 상품일 수 있습니다. 이러한 희소성은 만족스러운 성능을 달성하기 위해 일반적으로 수천 또는 수백만 개의 레이블이 지정된 예제가 필요한 기존의 지도 학습 접근 방식에 상당한 도전이 됩니다.
데이터 부족 문제는 희귀 질환으로 인해 문서화된 사례가 제한적인 의료 분야나 새로운 범주의 데이터가 자주 등장하는 급변하는 환경에서 특히 심각합니다. 이러한 시나리오에서는 대규모 데이터 세트를 수집하고 라벨을 지정하는 데 필요한 시간과 리소스가 엄청나게 많이 소요되어 AI 개발 및 배포에 병목 현상이 발생할 수 있습니다.
몇 번의 샷 학습 대 기존의 지도 학습
Understanding the distinction between Few Shot Learning and traditional supervised learning is crucial to grasp its real-world impact.
전통 지도 학습, while powerful, has drawbacks:
데이터 종속성: Struggles with limited training data.
유연성: Performs well only on specific trained tasks.
리소스 집약도: Requires large, expensive datasets.
지속적인 업데이트: Needs frequent retraining in dynamic environments.
Few Shot Learning offers a paradigm shift:
샘플 효율성: Generalizes from few examples using meta-learning.
빠른 적응: Quickly adapts to new tasks with minimal examples.
리소스 최적화: Reduces data collection and labeling needs.
지속적인 학습: Suitable for incorporating new knowledge without forgetting.
다용도성: Applicable across various domains, from computer vision to NLP.
By tackling these challenges, Few Shot Learning enables more adaptable and efficient AI models, opening new possibilities in AI development.
효율적인 샘플 학습의 스펙트럼
A fascinating spectrum of approaches aims to minimize required training data, including Zero Shot, One Shot, and Few Shot Learning.
제로 샷 학습: 예제 없이 학습하기
Recognizes unseen classes using auxiliary information like textual descriptions
Valuable when labeled examples for all classes are impractical or impossible
원샷 학습: 단일 인스턴스에서 학습하기
Recognizes new classes from just one example
Mimics human ability to grasp concepts quickly
Successful in areas like facial recognition
몇 번의 샷 학습: 최소한의 데이터로 작업 마스터하기
Uses 2-5 labeled examples per new class
Balances extreme data efficiency and traditional methods
Enables rapid adaptation to new tasks or classes
Leverages meta-learning strategies to learn how to learn
This spectrum of approaches offers unique capabilities in tackling the challenge of learning from limited examples, making them invaluable in data-scarce domains.
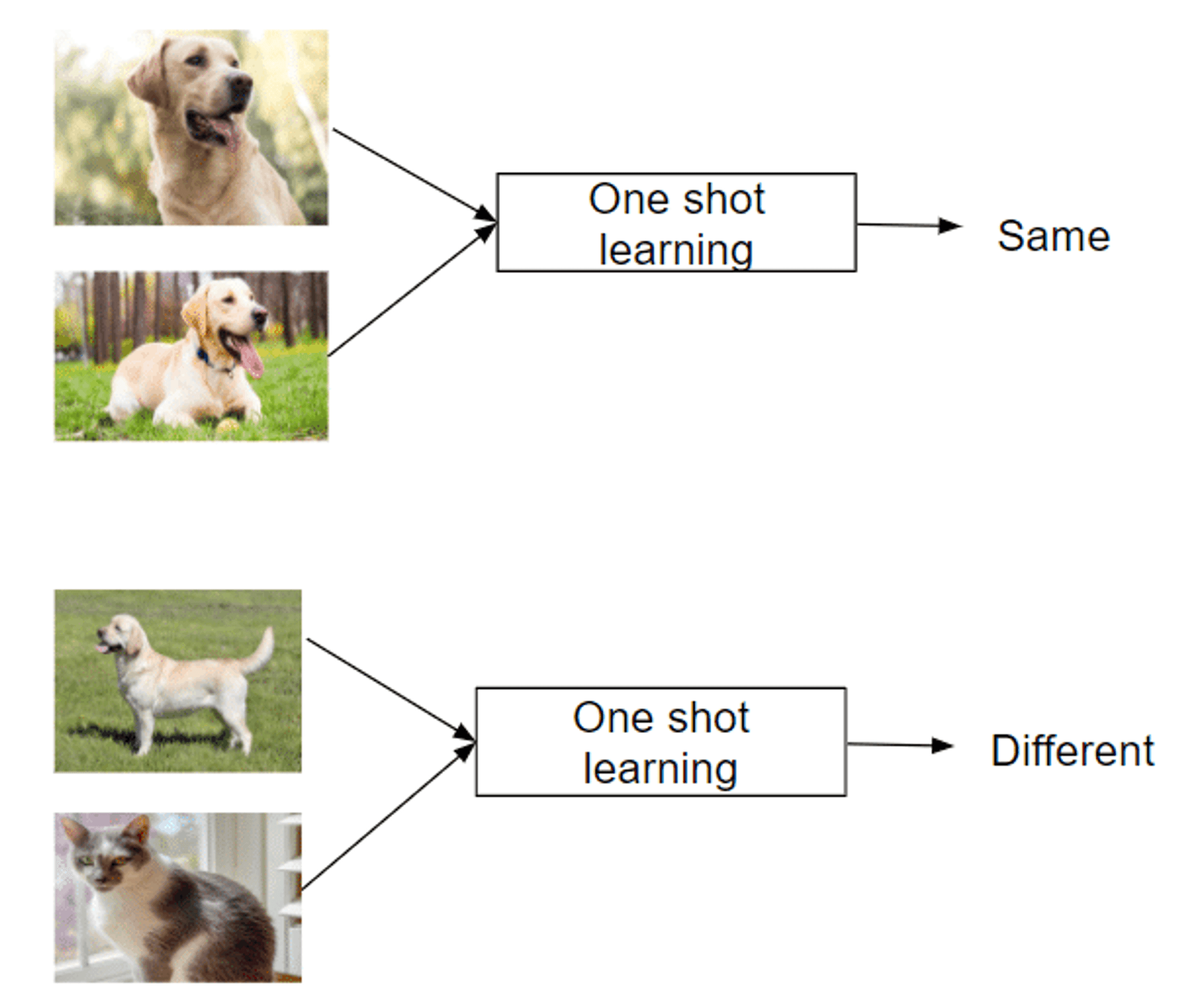
Few Shot Prompting vs Fine Tuning LLM
Two more powerful techniques exist in this realm: few-shot prompting and fine-tuning. Few-shot prompting involves crafting clever input prompts that include a small number of examples, guiding the model to perform a specific task without any additional training. Fine-tuning, on the other hand, involves updating the model’s parameters using a limited amount of task-specific data, allowing it to adapt its vast knowledge to a particular domain or application.
Both approaches fall under the umbrella of few-shot learning. By leveraging these techniques, we can dramatically enhance the performance and versatility of LLMs, making them more practical and effective tools for a wide range of applications in natural language processing and beyond.
몇 번만 촬영하면 됩니다: LLM 잠재력 발휘하기
Few-shot prompting capitalizes on the model’s ability to understand instructions, effectively “programming” the LLM through crafted prompts.
Few-shot prompting provides 1-5 examples demonstrating the desired task, leveraging the model’s pattern recognition and adaptability. This enables performance of tasks not explicitly trained for, tapping into the LLM’s capacity for in-context learning.
By presenting clear input-output patterns, few-shot prompting guides the LLM to apply similar reasoning to new inputs, allowing quick adaptation to new tasks without parameter updates.
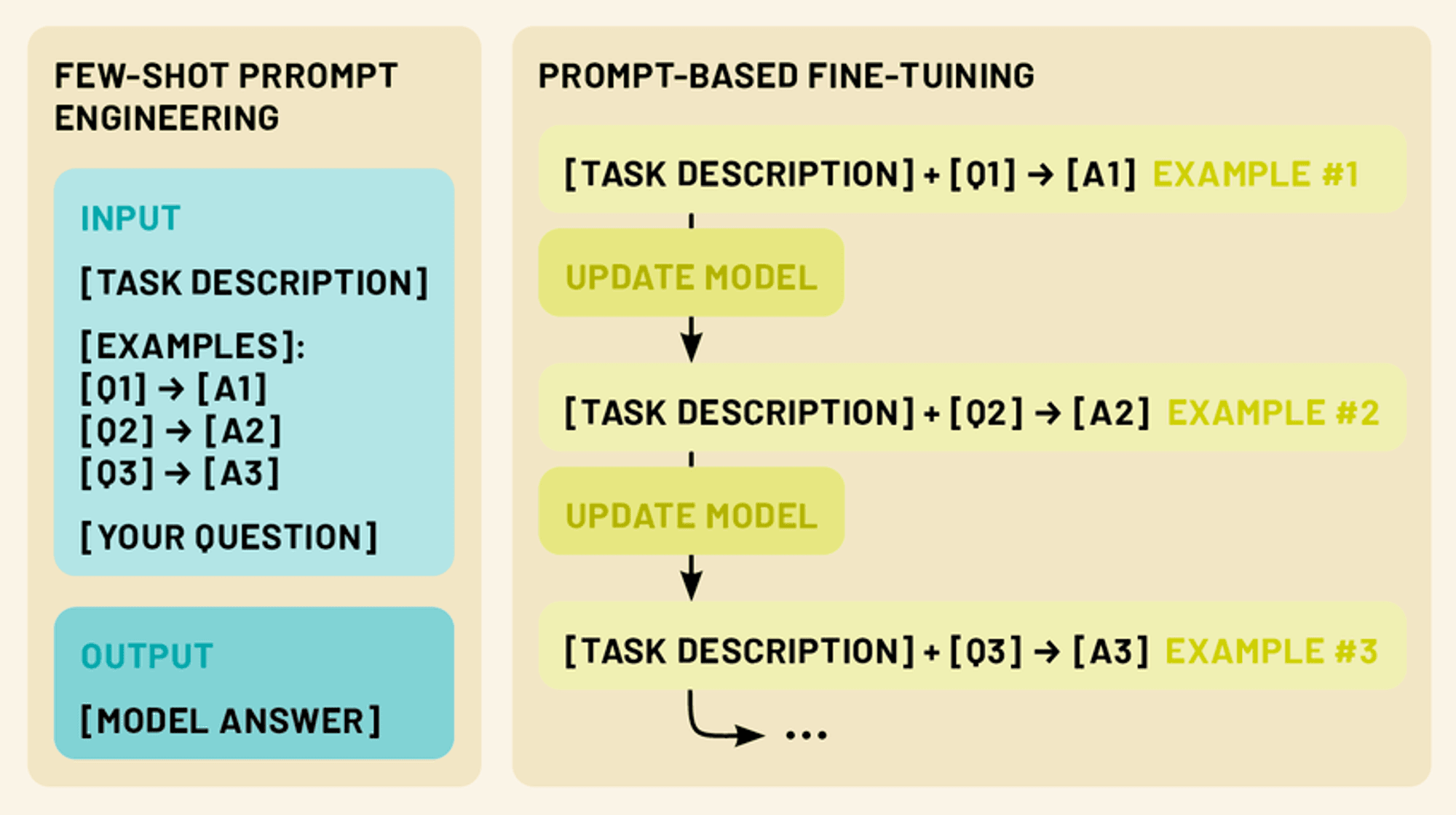
몇 샷 프롬프트 유형(제로 샷, 원샷, 몇 샷)
Few-shot prompting encompasses a spectrum of approaches, each defined by the number of examples provided. (Just like few-shot learning):
제로 샷 프롬프트: 이 시나리오에서는 예제가 제공되지 않습니다. 대신 모델에 작업에 대한 명확한 지침이나 설명이 제공됩니다. 예를 들어 "다음 영어 텍스트를 프랑스어로 번역: [입력 텍스트]"와 같은 예가 있습니다.
원샷 프롬프트: 여기에서는 실제 입력 전에 하나의 예가 제공됩니다. 이를 통해 모델에 예상되는 입력-출력 관계의 구체적인 인스턴스를 제공합니다. 예를 들어 "다음 리뷰의 감성을 긍정 또는 부정으로 분류하세요. 예: '이 영화는 환상적이었다!' - 긍정적 입력: '줄거리를 참을 수 없었다.' - [모델이 응답 생성]"
몇 번 촬영한 프롬프트: 이 접근 방식은 실제 입력 전에 여러 예제(일반적으로 2~5개)를 제공합니다. 이를 통해 모델은 작업에서 더 복잡한 패턴과 뉘앙스를 인식할 수 있습니다. 예를 들어 "다음 문장을 질문 또는 진술로 분류하세요: '하늘은 파랗다.' - 문 '지금 몇 시인가요?' - 질문 '나는 아이스크림을 좋아해요.' - 문 입력: '가장 가까운 식당을 어디에서 찾을 수 있나요?' - [모델에서 응답 생성]"
효과적인 단발성 프롬프트 디자인
효과적인 단발성 프롬프트를 만드는 것은 예술이자 과학입니다. 다음은 고려해야 할 몇 가지 주요 원칙입니다:
명확성과 일관성: 예제와 지침이 명확하고 일관된 형식을 따르도록 하세요. 이렇게 하면 모델이 패턴을 더 쉽게 인식하는 데 도움이 됩니다.
다양성: 여러 예제를 사용할 때는 가능한 입력과 출력의 범위를 포괄하여 모델이 작업을 더 폭넓게 이해할 수 있도록 하세요.
관련성: 타겟팅하는 특정 작업 또는 도메인과 밀접한 관련이 있는 예시를 선택하세요. 이렇게 하면 모델이 지식의 가장 관련성이 높은 측면에 집중할 수 있습니다.
간결함: 충분한 컨텍스트를 제공하는 것이 중요하지만, 모델을 혼란스럽게 하거나 핵심 정보를 희석시킬 수 있는 지나치게 길거나 복잡한 프롬프트는 피하세요.
실험: Don’t be afraid to iterate and experiment with different prompt structures and examples to find what works best for your specific use case.
단발성 프롬프트의 기술을 습득하면 최소한의 추가 입력이나 교육만으로 다양한 작업을 처리할 수 있도록 LLM의 잠재력을 최대한 발휘할 수 있습니다.
LLM 미세 조정: 제한된 데이터로 모델 조정하기
소량 프롬프트는 모델 자체를 수정하지 않고도 새로운 작업에 LLM을 적용할 수 있는 강력한 기술이지만, 미세 조정은 특정 작업이나 도메인에서 더 나은 성능을 위해 모델의 매개변수를 업데이트할 수 있는 방법을 제공합니다. 미세 조정을 사용하면 소량의 작업별 데이터만 사용하여 특정 요구 사항에 맞게 조정하면서 사전 학습된 LLM에 인코딩된 방대한 지식을 활용할 수 있습니다.
LLM의 맥락에서 미세 조정 이해하기
Fine-tuning an LLM involves further training a pre-trained model on a smaller, task-specific dataset. This process adapts the model to the target task while building upon existing knowledge, requiring less data and resources than training from scratch.
In LLMs, fine-tuning typically adjusts weights in upper layers for task-specific features, while lower layers remain largely unchanged. This “transfer learning” approach retains broad language understanding while developing specialized capabilities.
몇 번의 촬영으로 미세 조정하는 기술
Few-shot fine-tuning adapts the model using only 10 to 100 samples per class or task, valuable when labeled data is scarce. Key techniques include:
프롬프트 기반 미세 조정: Combines few-shot prompting with parameter updates.
메타 학습 접근 방식: Methods like MAML aim to find good initialization points for quick adaptation.
Adapter-based fine-tuning: Introduces small “adapter” modules between pre-trained model layers, reducing trainable parameters.
상황에 맞는 학습: Fine-tunes LLMs to better perform adaptation through prompts alone.
These techniques enable LLMs to adapt to new tasks with minimal data, enhancing their versatility and efficiency.
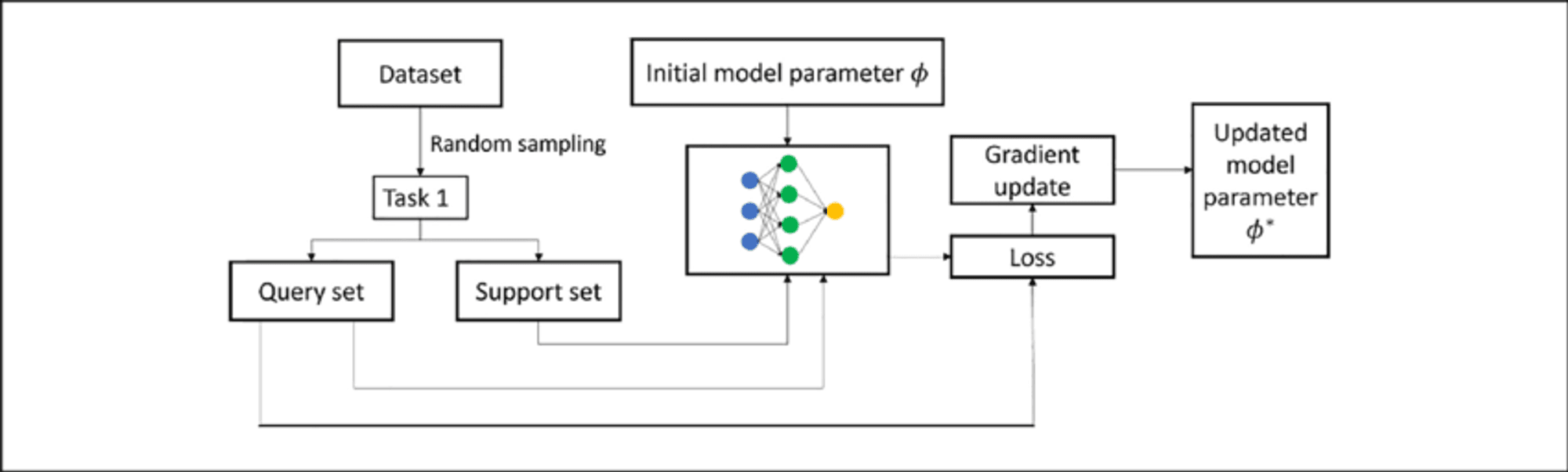
소수의 샷 프롬프트 대 미세 조정: 올바른 접근 방식 선택하기
특정 작업에 LLM을 적용하는 경우, 단발성 프롬프트와 미세 조정 모두 강력한 솔루션을 제공합니다. 그러나 각 방법에는 고유한 장점과 한계가 있으며 올바른 접근 방식을 선택하는 것은 다양한 요인에 따라 달라집니다.
Few-Shot Prompting Strengths:
모델 파라미터 업데이트가 필요하지 않으므로 원본 모델을 유지합니다.
유연성이 뛰어나며 즉시 적용 가능
추가 교육 시간이나 컴퓨팅 리소스가 필요하지 않습니다.
빠른 프로토타이핑 및 실험에 유용합니다.
제한 사항:
특히 복잡한 작업의 경우 성능이 일관성이 떨어질 수 있습니다.
모델의 원래 기능과 지식에 의해 제한됨
고도로 전문화된 도메인이나 작업에서 어려움을 겪을 수 있습니다.
Fine-Tuning Strengths:
특정 작업에서 더 나은 성과를 달성하는 경우가 많습니다.
새로운 도메인 및 전문 어휘에 맞게 모델 조정 가능
유사한 입력값에 대해 보다 일관된 결과 제공
지속적인 학습 및 개선 가능성
제한 사항:
추가 교육 시간 및 컴퓨팅 리소스 필요
주의 깊게 관리하지 않으면 치명적인 망각의 위험이 있습니다.
소규모 데이터 세트에 과대 적합할 수 있음
유연성 저하, 중요한 작업 변경 시 재교육 필요
Top 5 Research Papers for Few-Shot Learning
This week, we also explore the following five papers that have significantly advanced this field, introducing innovative approaches that are reshaping AI capabilities.
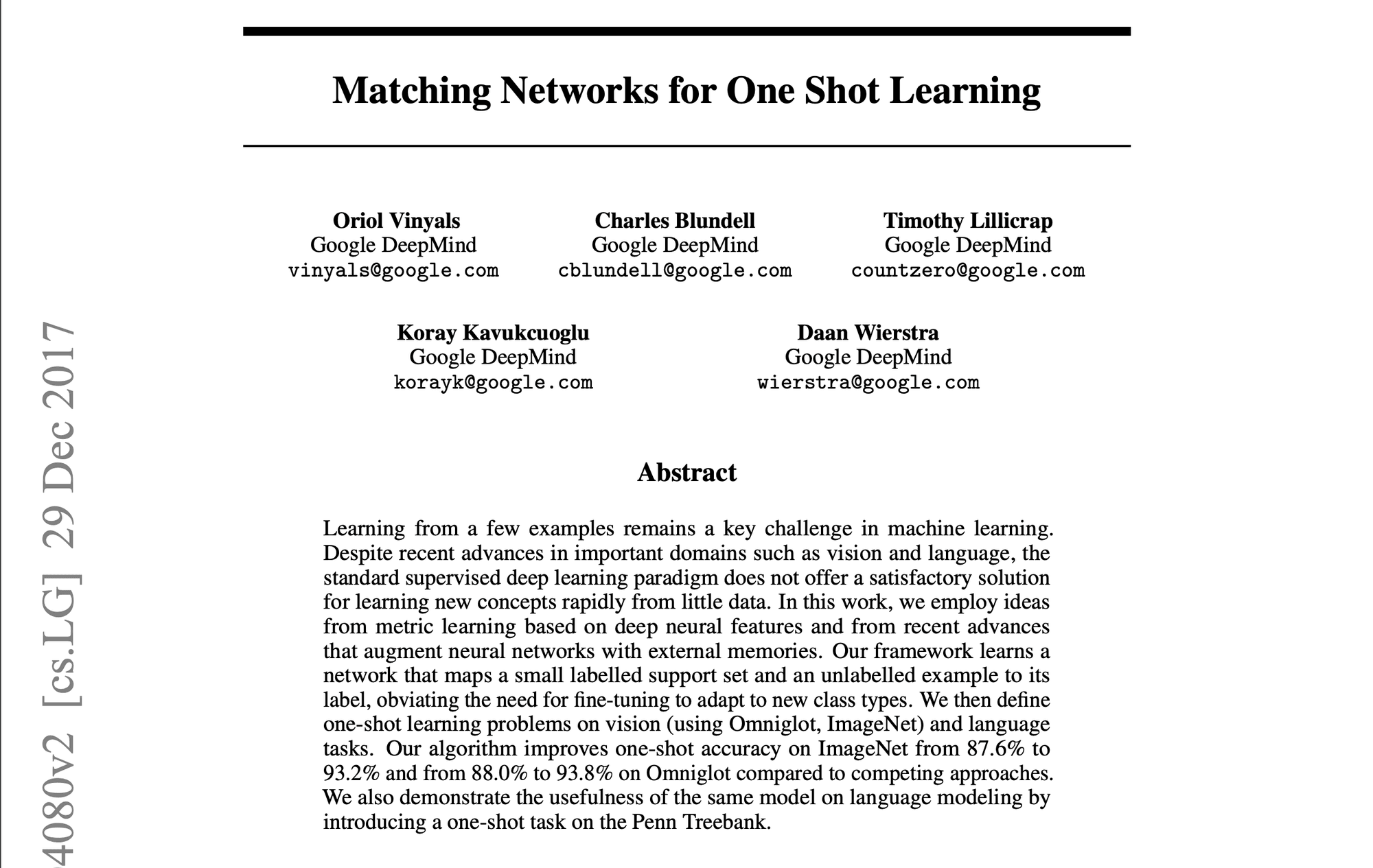
1️⃣ Matching Networks for One Shot Learning” (Vinyals et al., 2016)
Introduced a groundbreaking approach using memory and attention mechanisms. The matching function compares query examples to labeled support examples, setting a new standard for few-shot learning methods.
2️⃣ Prototypical Networks for Few-shot Learning” (Snell et al., 2017)
Presented a simpler yet effective approach, learning a metric space where classes are represented by a single prototype. Its simplicity and effectiveness made it a popular baseline for subsequent research.
3️⃣ Learning to Compare: Relation Network for Few-Shot Learning” (Sung et al., 2018)
Introduced a learnable relation module, allowing the model to learn a comparison metric tailored to specific tasks and data distributions. Demonstrated strong performance across various benchmarks.
4️⃣ A Closer Look at Few-shot Classification” (Chen et al., 2019)
Provided a comprehensive analysis of existing methods, challenging common assumptions. Proposed simple baseline models that matched or exceeded more complex approaches, emphasizing the importance of feature backbones and training strategies.
5️⃣ Meta-Baseline: Exploring Simple Meta-Learning for Few-Shot Learning” (Chen et al., 2021)
Combined standard pre-training with a meta-learning stage, achieving state-of-the-art performance. Highlighted the trade-offs between standard training and meta-learning objectives.
These papers have not only advanced academic research but also paved the way for practical applications in enterprise AI. They represent a progression towards more efficient, adaptable AI systems capable of learning from limited data – a crucial capability in many business contexts.
결론
Few-shot learning, prompting, and fine-tuning represent groundbreaking approaches, enabling LLMs to adapt swiftly to specialized tasks with minimal data. As we’ve explored, these techniques offer unprecedented flexibility and efficiency in tailoring LLMs to diverse applications across industries, from enhancing natural language processing tasks to enabling domain-specific adaptations in fields like healthcare, law, and technology.
시간을 내어 AI & YOU를 읽어주셔서 감사합니다!
인포그래픽, 통계, 방법 가이드, 기사, 동영상 등 엔터프라이즈 AI에 관한 더 많은 콘텐츠를 보려면 다음에서 Skim AI를 팔로우하세요. LinkedIn
AI 자문, 부분적인 AI 개발 또는 실사 서비스를 찾고 있는 창업자, CEO, 벤처 캐피털리스트 또는 투자자이신가요? 귀사의 AI 제품 전략 및 투자 기회에 대해 정보에 입각한 결정을 내리는 데 필요한 가이드를 받아보세요.
엔터프라이즈 AI 솔루션을 시작하는 데 도움이 필요하신가요? 유니티의 AI 워크포스 관리 플랫폼으로 나만의 AI 워커를 구축하고 싶으신가요? 상담 신청하기
유니티는 다음 산업 분야의 벤처 캐피탈 및 사모펀드 지원 기업을 위한 맞춤형 AI 솔루션을 구축합니다: 의료 기술, 뉴스/콘텐츠 집계, 영화 및 사진 제작, 교육 기술, 법률 기술, 핀테크 및 암호화폐.